AI for ROI: Methods to Guarantee Optimal Returns from Your AI Investment
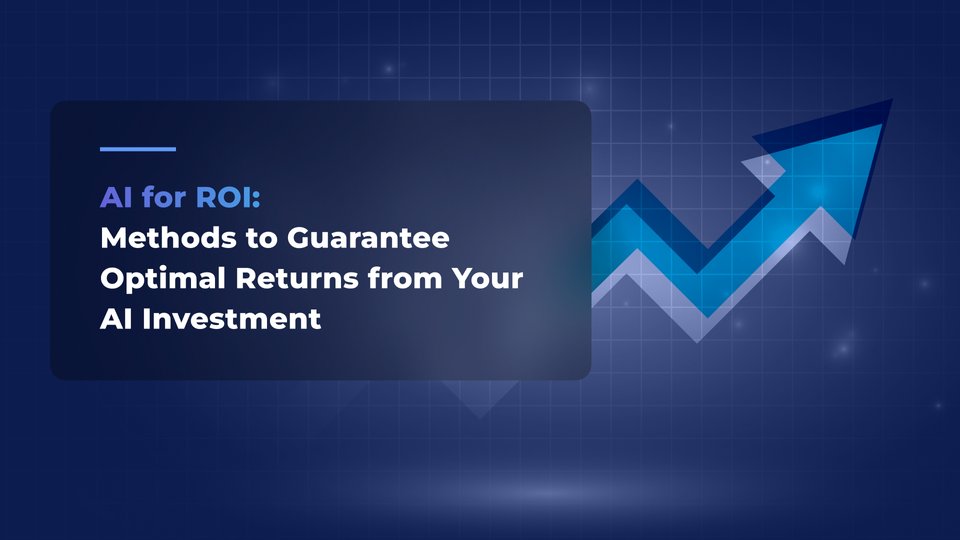
Many IT leaders find themselves in a tough spot when trying to get approval for AI projects from their CFOs. The big question they face is: How do you calculate the return on investment (ROI) for AI? It’s tricky because while AI has immense potential, its benefits often show up indirectly and over a longer period. This makes it hard to present a compelling ROI case to CFOs who have to prioritize immediate financial returns.
An IBM study reveals promising results: An IBM study reveals promising results: companies that strategically deploy artificial intelligence achieve an average 13% return on their projects — more than double the typical ROI of 5.9%. Additionally, another study found that for every dollar invested in artificial intelligence, companies can expect an average return of $3.50.
At Flyaps, we’ve seen firsthand how artificial intelligence transforms businesses. As a software development company with a proven record in AI implementation, we’ve helped organizations across sectors, from human resources to urban planning, leverage this technology to meet their business goals. This article breaks down how to measure and achieve ROI with AI, turning it into a source of tangible results. Keep reading to discover six steps to double your ROI from AI investments.
Steps to achieve impressive AI ROI
Maximizing the ROI of AI starts with a solid strategy and hinges on six key steps, outlined below.
Step 1. Identify where AI investments can be put to the best possible use
Artificial intelligence is often seen as a transformative technology that helps businesses streamline operations and improve efficiency. However, it’s not always the best tool for every task. For instance, if you’re looking to automate invoicing processes or enhance customer support, the choice of technology matters.
While AI tools can handle both areas effectively, robotic process automation (RPA) might be a more cost-effective and faster option for invoicing. RPA excels at automating repetitive tasks like entering invoice data into systems. It’s simple to implement, doesn’t demand extensive data training, and delivers quick wins by reducing manual processes.
On the other hand, for improving customer support, AI technologies take the lead. Specifically, a large language model (LLM) — a type of generative AI — works well for building chatbots that handle common customer questions. These models generate human-like responses, enhancing customer experience and streamlining workflows.
An AI strategy should align with clear business objectives, not just trends. Research by IBM shows that organizations integrating this technology into their business strategies effectively are 1.8 times more likely to achieve the double ROI we talked about earlier.
Ultimately, knowing where and how artificial intelligence can impact your operations is crucial. With a clear approach, you're much more likely to see tangible benefits, reduce operational costs, and maximize the return on your AI investments.
Step 2. Choose an AI operating model
Once you identify where to implement artificial intelligence, the next step is selecting an AI operating model — a framework for developing, deploying, and managing AI. Just as teams in sports adopt different strategies, businesses need to decide on the best approach for integrating AI based on their specific business objectives and resources.
There are three main types of AI operating models:
1. Centralized
Artificial intelligence initiatives are managed by a single, central team. For instance, a multinational bank’s central AI team oversees its entire portfolio of AI projects, from fraud detection to customer service chatbots. This team sets the standards, develops the AI tools, and ensures that each AI project aligns with the bank’s business objectives and compliance needs.
2. Hub-and-spoke
A central team (hub) collaborates with decentralized teams within different departments (spokes). Suppose, the bank's central artificial intelligence team develops core tools and frameworks. Each department, such as retail, investment, and compliance, has its own specialists (spokes) who adapt these tools to their unique needs. For instance, the retail team might focus on enhancing customer experience with generative AI-powered personalization tools, while the compliance team uses artificial intelligence to monitor transactions for suspicious activity. This way, the central team ensures strategic alignment and provides support, while the departmental teams tailor the AI solutions to their specific requirements.
3. Decentralized
AI projects are managed independently within each business unit or department. In this case, each department within the bank operates its own artificial intelligence initiatives without central coordination. In our imaginary example, the retail department would develop its own tools for customer behavior analysis and personalized banking recommendations while the corporate banking division would be busy creating separate artificial intelligence models to predict market trends and investment opportunities. While this operating model lets each department tackle their own challenges, it can also lead to wasting resources and duplicated efforts. For instance, different teams might work on similar projects, like developing their own fraud detection algorithms.
Step 3. Consider following the artificial intelligence operations (AIOps) approach
AIOps is a practice to enhance and streamline the management of IT systems using AI. Basically, if you combine Agile DevOps, MLOps, and automated ITOps with AI you will have AIOps. The goal of this approach is to deploy AI solutions that are flexible, user-friendly, and scalable. To do this, AIOps incorporates three crucial elements:
- Skilled teams who develop and manage solutions.
- Structured processes that ensure projects are developed and deployed efficiently.
- The technology and tools that support AI applications.
Together, these elements help organizations apply AI quickly and on a large scale.
When it comes to achieving better ROI with AI, AIOps is aligning AI operating models to ensure that IT operations are managed consistently across different departments. For example, in a hub-and-spoke model, the central tech team could set up the core AIOps infrastructure and tools and the departmental teams would then customize these tools to meet their specific needs.
Step 4. Automate and protect your data
Data is the lifeblood of AI. No other technology depends as deeply on data for its success. The way a company manages its data can make or break the success of its projects and directly impact the ROI.
Here’s how you can make sure your data helps you get the most out of your AI initiatives:
1. Set up systems where your team can quickly access the data they need. For example, use a centralized data platform where all your important information is stored in one place.
2. Automate your data collection and cleaning processes to save time and ensure consistency. For instance, opt for tools like Trifacta Wrangler or Astera Centerprise to automatically gather needed data from different sources and clean it up, so it’s ready for AI to analyze right away.
3. Implement systems that continuously monitor and fix data errors in real-time, ensuring your AI models are always working with the best possible information.
4. Use encryption and strict access controls to make sure that only the right people can see or use sensitive data.
You can read more about data management for AI in our article “10 Principles to Create a Strong Data Foundation for AI Success.”
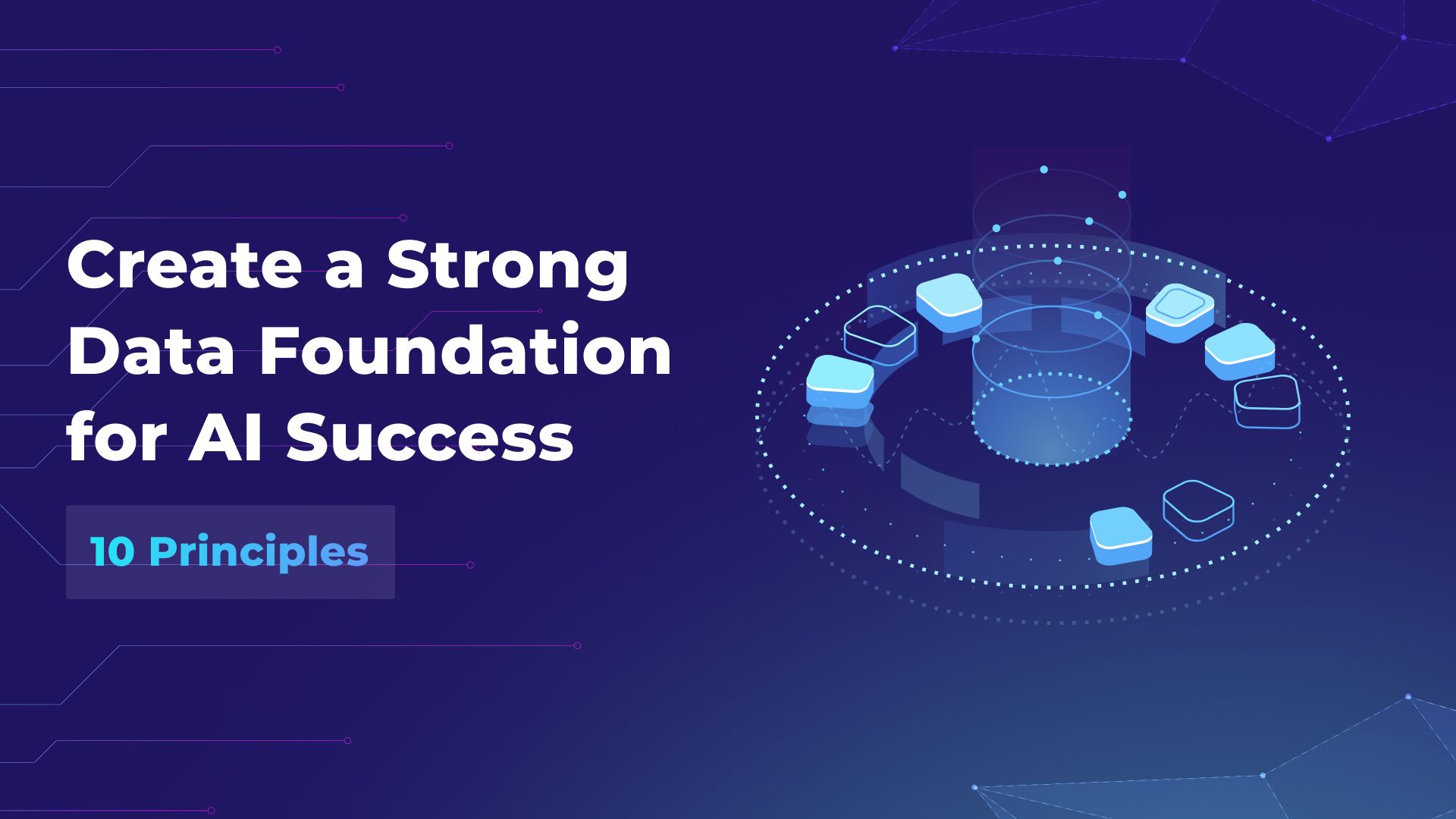
Step 5. Invest in a skilled workforce
According to Upwork, job postings for generative artificial intelligence skyrocketed by over 1000% in the second quarter of 2023, highlighting the growing role of artificial intelligence talent and skills and their impact on ROI.
Start by defining the roles essential for developing and maintaining your project.such as machine learning specialists, data engineers ensuring data quality, and others.
For a full breakdown, check out our article “9 Generative AI Roles You Need for Your Business.”
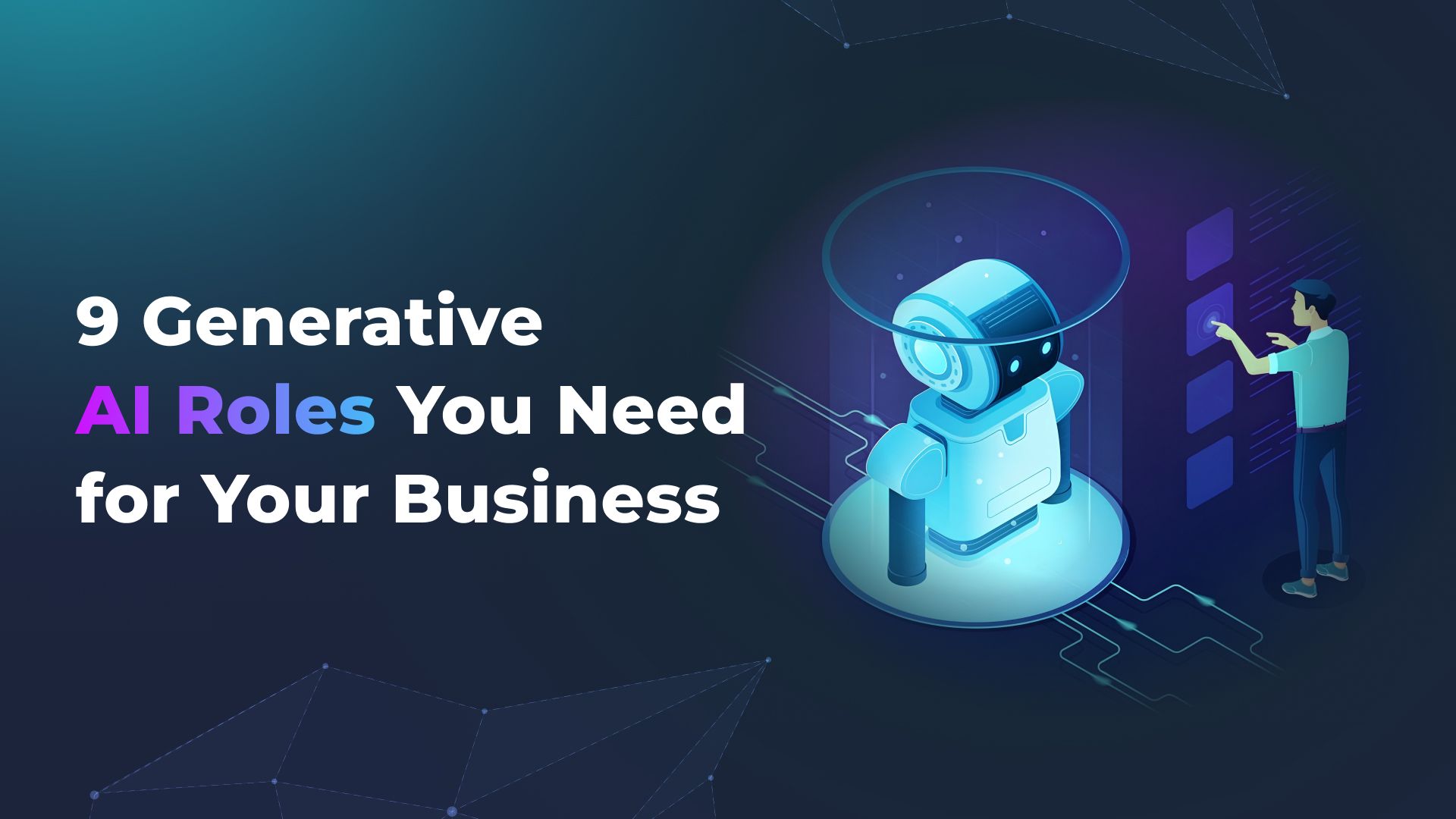
Continuous learning keeps employees up-to-date with the advancements, enabling them to apply new techniques and tools effectively. Additionally, offer no-code or low-code tools to simplify artificial intelligence development.
Step 6. Be open for change and co-creation
The real value of any AI system unfolds only when employees and stakeholders trust such tools. The adoption of this technology isn't always smooth sailing. Think back to when the Internet just became mainstream. Many were skeptical, but today, it's an integral part of our lives. Similarly, for AI to be embraced fully, employees and stakeholders must see its business outcomes and believe it’s worth the investment.
To build this trust and drive adoption, you can take several steps:
- Launch small, manageable projects to test the waters. Treating each AI project individually allows teams to understand the technology’s potential and build confidence before rolling out larger initiatives. For instance, a small marketing firm can start with an email campaign tool that analyzes historical data and recommends optimized subject lines and personalized recommendations for improving the open rate. If the tool boosts open rates and enhances employee productivity, the team will be convinced of AI's capabilities.
- Use tools like ChatGPT during meetings or brainstorming sessions to showcase its practical benefits and encourage others to get on board.
- Identify and support enthusiastic employees who can advocate for AI. Suppose, a tech company provided a software engineer passionate about this technology with additional training and resources to deepen their knowledge of this technology. Over time, the engineer may start holding workshops for their colleagues, helping to align business teams with the organization’s AI journey.
Key KPIs to calculate ROI with AI
KPIs can be direct, such as cost savings from automation or revenue increases from AI-driven marketing campaigns. They can also be indirect, capturing qualitative benefits like improved decision-making or competitive advantage.
Examples of direct KPIs:
Cost savings. Measures reductions in labor costs, improved operational efficiency, or optimized resource utilization achieved through AI automation.
Revenue increase. Lets you track gains in revenue from enhanced customer acquisition, cross-selling, or personalized recommendations driven by AI insights.
Marketing effectiveness. Helps evaluate metrics such as cost per acquisition (CPA), conversion rates, or return on advertising spend (ROAS) from AI-powered marketing campaigns.
Examples of indirect KPIs:
Improved decision-making. Suppose, a retail company uses AI to analyze vast amounts of sales and customer data, leading to more informed and strategic choices in inventory management and marketing tactics. This aligns with business objectives, resulting in better market responsiveness and more effective promotional campaigns, even if the financial ratio of the impact isn’t immediately obvious.
Competitive advantage. For instance, a logistics company integrates AI strategies to optimize route planning and delivery schedules. While direct benefits like reduced fuel costs are apparent, the long-term business outcomes gained through faster delivery times and improved customer service position the company as a market leader, attracting more clients, new partnerships, and building revenue streams over time.
Though indirect business factors will definitely influence your AI’s ROI, they are challenging to calculate. With that said, let’s look at how to calculate direct KPIs.
AI ROI calculation formula
Basically, you can calculate the AI ROI in three steps:
- Start by determining how much money the project has saved by reducing or eliminating certain expenses. This could be from automation, improved efficiency, or reduced errors.
- Next, measure how much additional income the project has generated. This might come from new sales, enhanced customer acquisition, or other revenue-driving activities enabled by AI.
- Finally, add up all the expenses related to the project over its entire lifecycle. This includes initial setup costs, ongoing operational expenses, maintenance, and any other costs associated with running the AI solution.
Once you have these values, plug them into the formula: ROI = cost savings + revenue – total cost of ownership (TCO).
Let’s say a company implements a system that:
1. Saves $200,000 annually by automating repetitive tasks (cost savings).
2. Generates an additional $300,000 in revenue through improved customer insights (revenue).
3. Has a total cost of ownership of $250,000 over its operational period (TCO).
Plugging these values into the formula: $200,000 + $300,000 - $250,000 = $250,000
In this case, the ROI is $250,000, meaning the project has generated $250,000 after accounting for the costs of implementation and operation.
Real-world example: how GitHub Copilot enhances ROI with AI
$21 per user per month can be a significant investment if you have a big team of developers. That is exactly how much GitHub Copilot’s enterprise plan costs.
GitHub Copilot is a generative AI tool designed by GitHub to assist developers in writing code. But according to Kyle Daigle, GitHub's Chief Operating Officer, the vision behind Copilot goes beyond simply assisting with code generation. The goal is to help developers build impactful projects more efficiently, driving broader human progress. Copilot reduces the time to market for new features and products by accelerating development cycles. This time efficiency translates into cost savings and revenue growth. But let’s do some math.
Suppose, the average cost of a software developer is $120 per hour and you have a team of 10 developers using GitHub Copilot. Let's assume a developer works 160 hours per month (40 hours per week). The monthly cost for 1 developer will be $19,200 (160×$120). Monthly cost for 10 developers - $192,000 (10×$19,200).
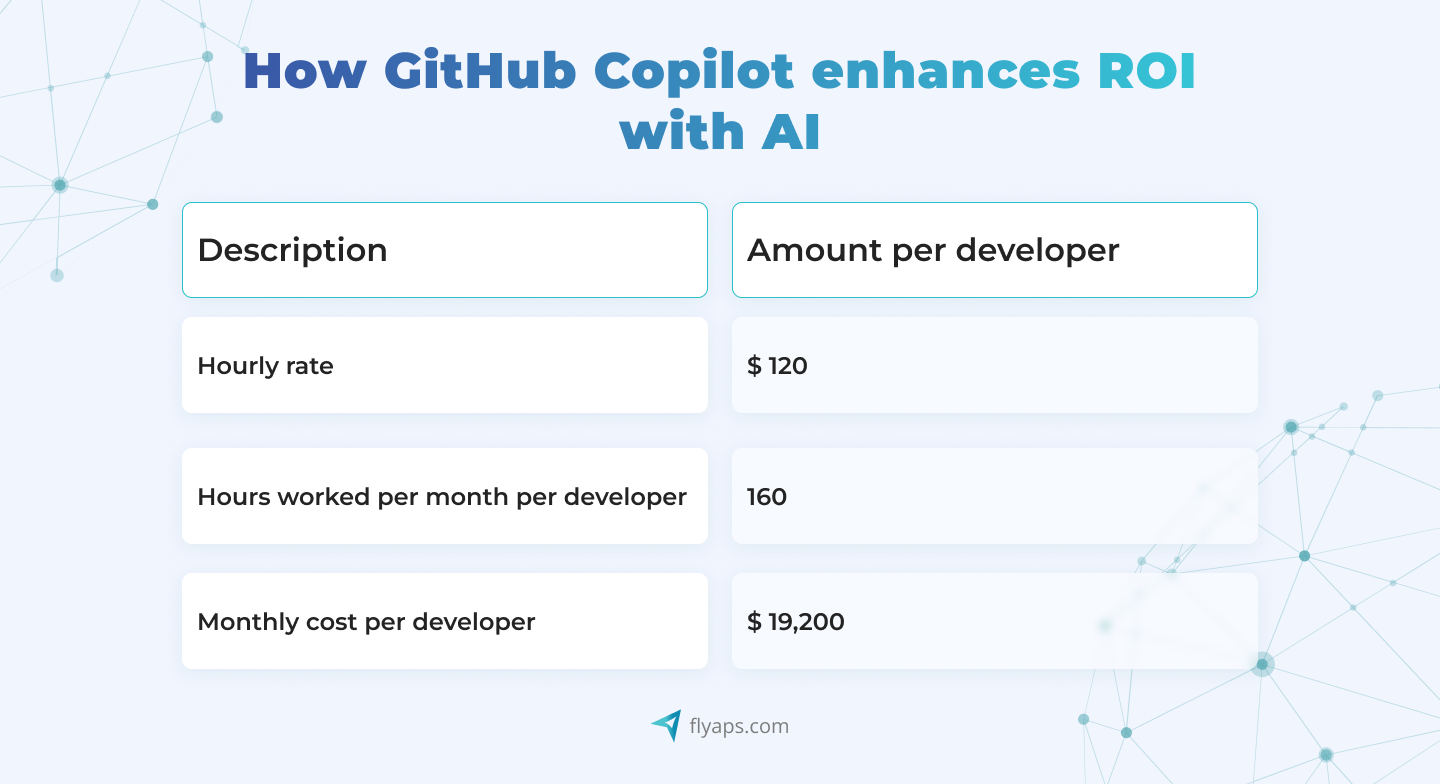
According to GitHub's research, their Copilot helps developers write code 55% faster. When developers are 55% faster, they complete tasks in 45% of the time. With the productivity boost, each developer effectively works 72 hours to produce the same amount of work (160×0.45). The monthly cost per developer using Copilot will be $8,640 (72×$120), and the monthly cost for 10 developers will be $210 (10×$21).
The resulting monthly savings will be $105,600 ($192,000-$86,400). The monthly cost of Copilot for 10 developers - $210 (10×$21). Finally, the net monthly savings is $105,390 ($105,600-$210).
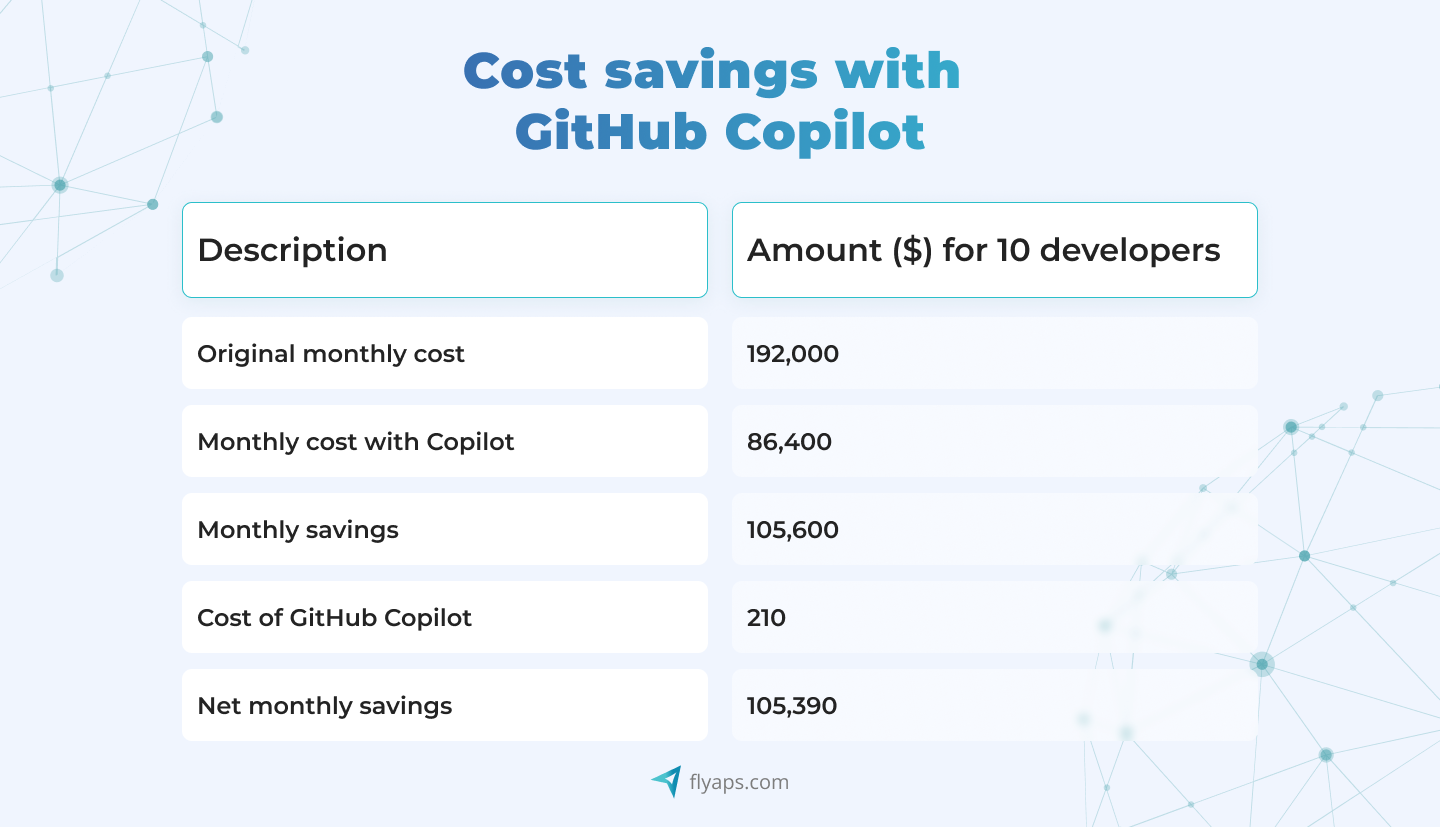
While it's tough to put a number on the improved job satisfaction and well-being that GitHub Copilot as an AI tool brings, the concrete financial benefits are clear as day. Therefore, by looking at the direct savings and increased efficiency, companies can see immediate returns on their investment in AI. Not only that, they can also forecast future financial gains from adopting AI tools like this.
Achieve higher ROI AI with Flyaps today
At Flyaps, we craft artificial intelligence solutions tailored to your business goals and financial ambitions. Our approach goes beyond solving immediate challenges—we design tools that serve as long-term assets. Take CV Compiler: a tool we developed that not only streamlined processes for our client but also gained traction among businesses tackling similar obstacles.
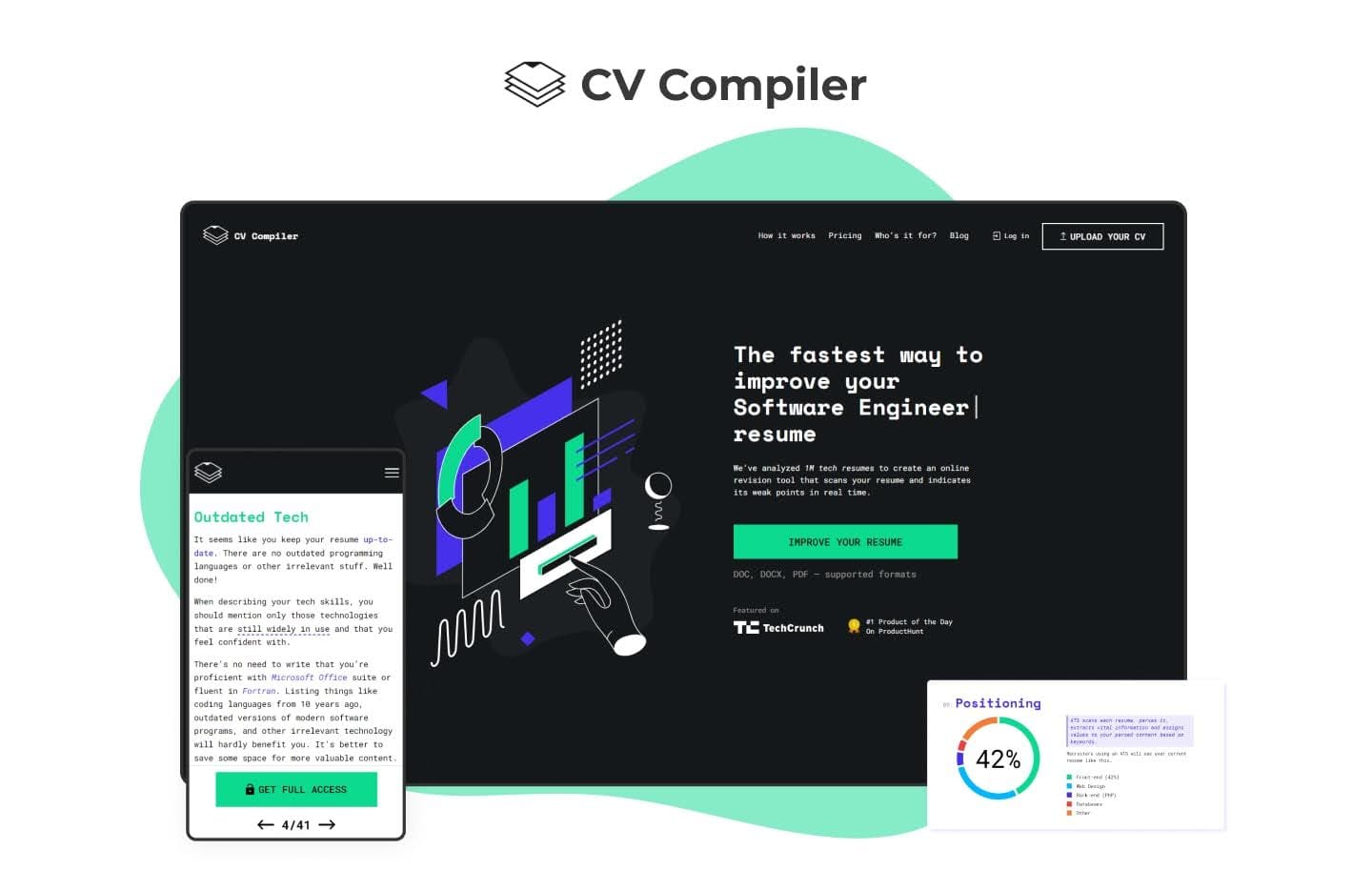
We also offer a selection of pre-built AI tools that integrate seamlessly with your systems. These solutions deliver the advantages of artificial intelligence without the significant costs of building from scratch.
Looking for an experienced team that focused on tackling current challenges and equipping you with cutting-edge tools for the future? Drop us a line!
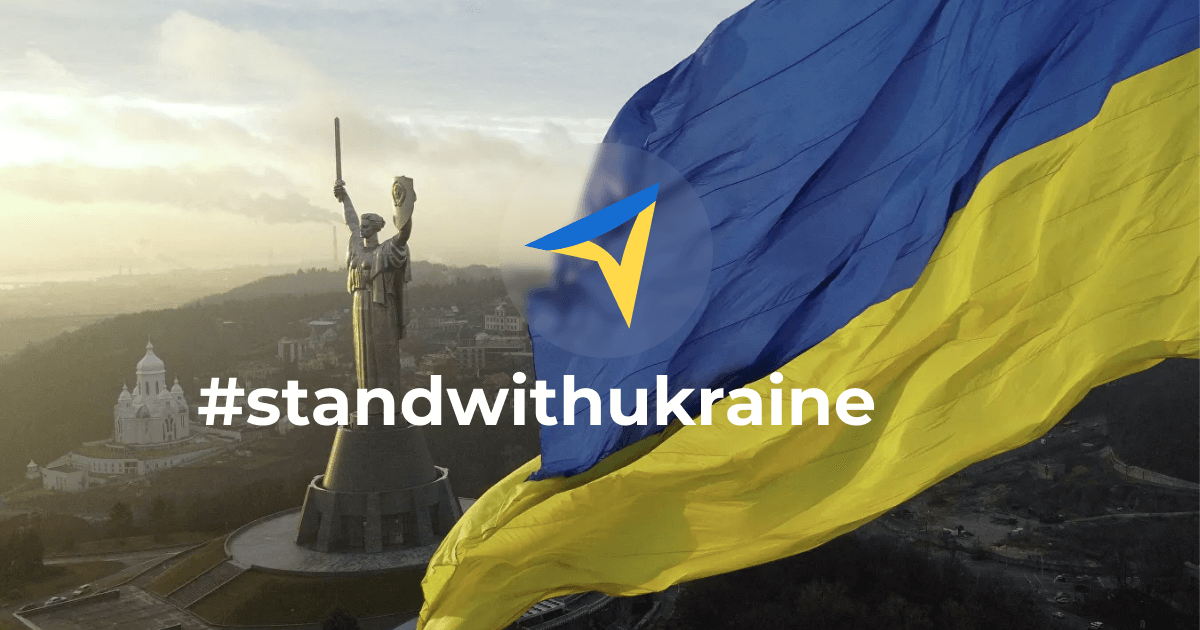