10 Principles to Create a Strong Data Foundation for AI Success
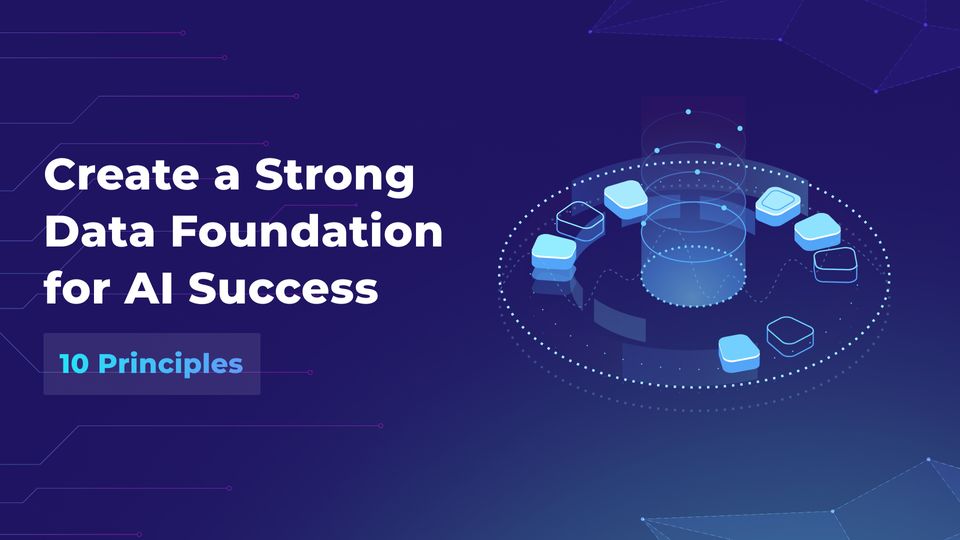
The excitement around generative AI is hard to miss, especially in the tech domain. With companies eager to stay ahead, the rush to adopt this technology of the future is palpable. But, a word of caution: diving headfirst into AI implementation without a second thought is not the wisest move. Why, you ask? Well, it turns out the key challenge lies in the data foundation for AI — specifically, a shortage of the right data for accurate model training and concerns over data security and privacy.
At Flyaps, an AI-focused software development company from NY, we understand the critical role data plays in AI. We've witnessed numerous cases in the industry where the lack of quality or quantity in data blocked the realization of brilliant ideas. Conversely, we've seen how strategic data collection and preparation can propel AI to success. Take, for instance, the case of GlossaryTech extension and CV Compiler, where we successfully managed the data aspect to create innovative AI-driven solutions for our clients. Join us as we delve into 10 proven principles that form the bedrock for building a robust data foundation for AI-driven applications.
The role of data quality for AI
Data is the heartbeat of any AI endeavor. Every decision an AI-driven product makes, every prediction it offers, stems from the patterns and insights it uncovers within the vast sea of data it has been exposed to. It's not just about quantity, but also quality, the richness of the information that molds intelligence.
First of all, AI models have to be trained with a discerning eye for data quality. Think of it as the clarity of a lens through which an AI perceives the world. If the lens is clouded or distorted, the AI's vision — its ability to understand, interpret, and respond — becomes compromised.
When we speak of data quality, we're referring to the accuracy, reliability, and relevance of the information at the AI model's disposal. Just as a reliable compass guides a traveler through uncharted territories, high-quality data steers AI models toward more precise and trustworthy outcomes.
Consequences resulting from a weak data foundation
Low-quality data can have far-reaching consequences, permeating through various aspects of AI applications and decision-making processes. Some notable consequences include the following:
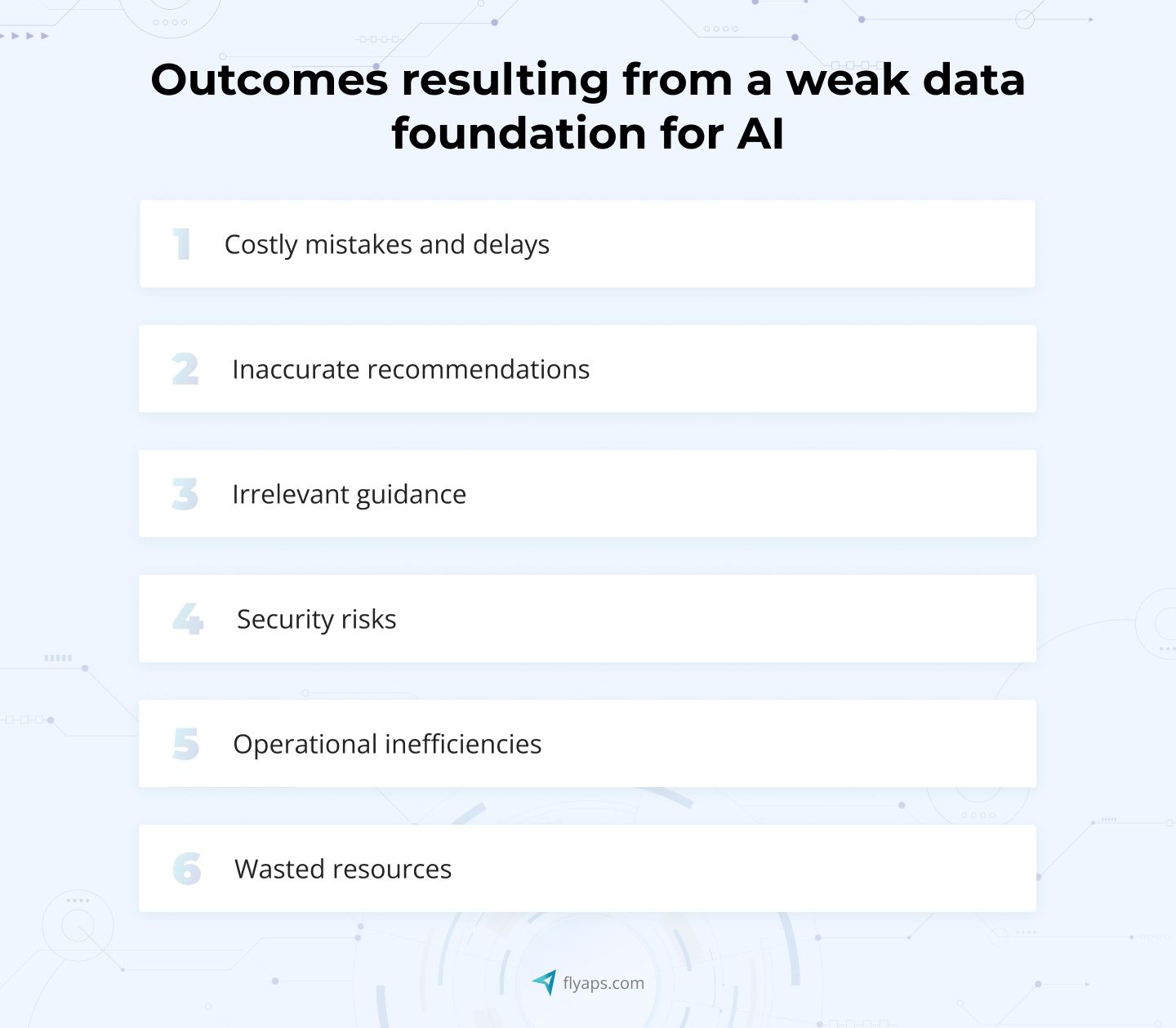
- Costly mistakes and delays: Without a solid data foundation, AI projects are susceptible to costly mistakes and delays. Inaccuracies in the data used for training can lead to flawed models, resulting in errors and setbacks in decision-making.
For example, think of a financial institution implementing an AI-driven fraud detection system that relies on inaccurate historical transaction data. When the model fails to accurately identify fraudulent activities it leads to delayed responses and significant financial losses.
- Inaccurate recommendations: Poor-quality data foundation for AI can lead to wrong recommendations. Whether it's suggesting products to users or making decisions based on faulty data, the outcomes may fall short of expectations and user needs.
Suppose an e-commerce platform, using flawed customer preference data, suggests products that do not align with users' actual interests. Customers receive irrelevant recommendations, resulting in decreased satisfaction, lower sales, and potential loss of customer trust.
- Irrelevant guidance: When data is outdated, the guidance provided by AI systems may become pointless in the current context. This can impact the applicability and effectiveness of AI-driven solutions.
For instance, let’s say a supply chain management company employs an AI system for inventory forecasting. However, if the data used for forecasting lacks relevance, the system may recommend stock levels based on older trends, leading to overstocking or stockouts and disrupting operational efficiency.
- Security risks: Inadequate data quality can introduce security risks. Flawed data may lead to vulnerabilities in AI systems, potentially compromising sensitive information and exposing organizations to security threats.
For instance, if an autonomous vehicle manufacturer incorporates AI algorithms trained on compromised sensor data, the security vulnerabilities in the data could lead to incorrect decisions by the vehicle, posing risks of accidents or malicious manipulation of the system.
- Operational inefficiencies: Low-quality data can lead to operational inefficiencies. AI systems reliant on accurate or complete data may need help to perform optimally, leading to suboptimal resource utilization and operational processes.
For example, a manufacturing company implements AI-driven predictive maintenance based on incomplete equipment sensor data. The AI system fails to predict maintenance needs precisely, resulting in unplanned downtime, increased maintenance costs, and disruptions to production schedules.
- Wasted resources: Lastly, investing time and resources in training AI models with low-quality data can be counterproductive. The results might be wasted efforts and resources, with the need for reiterations and corrections to address the shortcomings in the data.
Let’s suppose a technology firm invested significant resources in developing an AI-powered customer support chatbot. However, if the training data is of low quality, the chatbot may provide incorrect information, leading to customer dissatisfaction and necessitating additional resources for corrections and improvements.
So it’s evident that data is crucial for AI, and if it's not prepared well, the outcomes can be harmful to your business operations. With that being said, let's now explore the essential steps of getting data ready for AI in the next paragraph.
Steps of preparing a solid data foundation for AI
Here are some strategic tips to ensure that your data foundation paves the way for successful generative AI implementation and drives positive business outcomes:
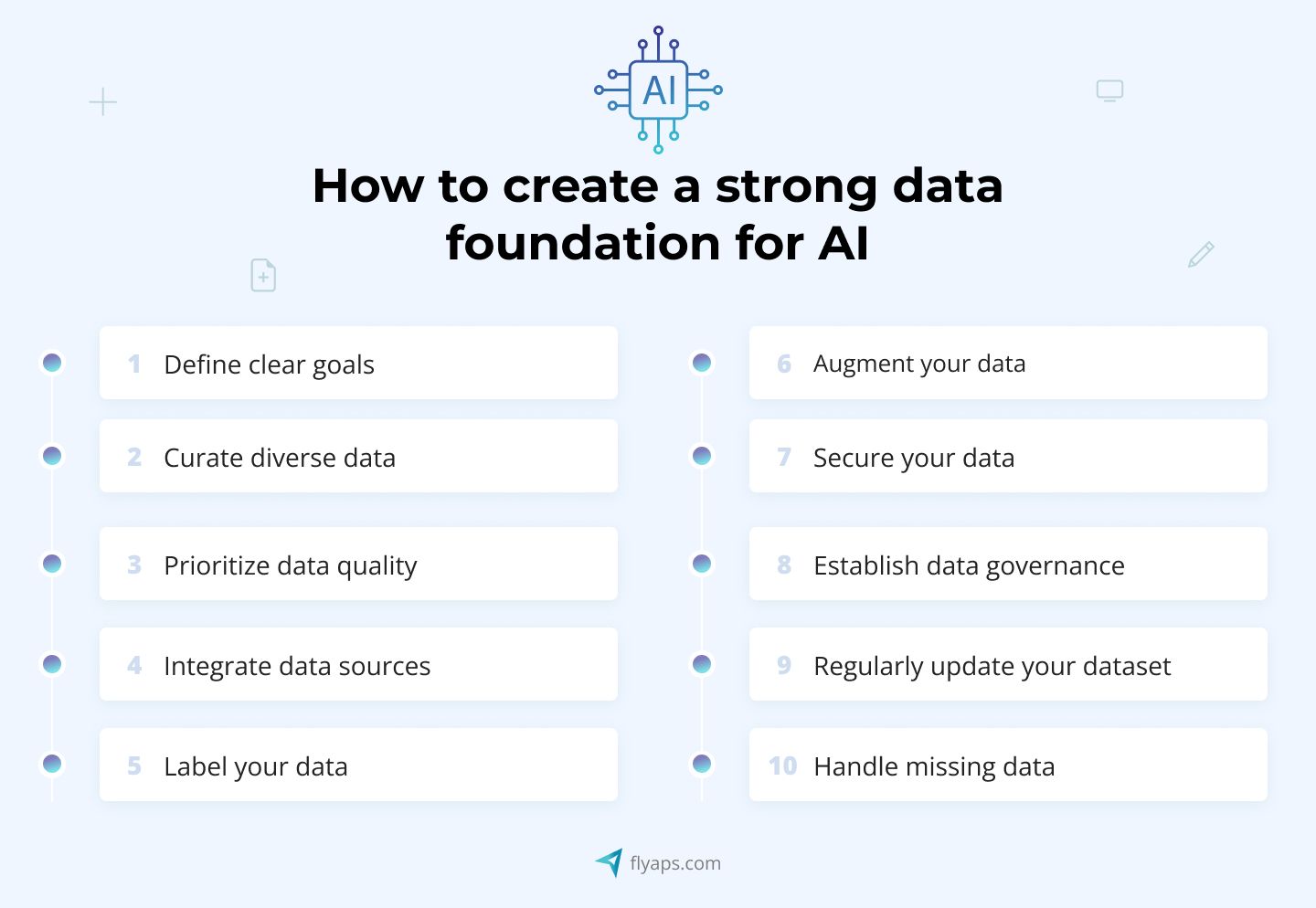
1. Define clear goals
Before diving into generative AI, identify your project goals. Define specific business challenges or opportunities you intend to address. With clear goals underlined, you’ll be able to come up with a roadmap for effective implementation.
2. Curate diverse data
Gather a diverse set of data relevant to your business objectives. Ensure that your dataset encompasses a variety of scenarios and contexts, allowing the generative AI model to comprehend and generate outputs that reflect real-world complexity.
As an example, an e-commerce platform has to gather diverse data, including customer purchase history, browsing behavior, and demographic information. This varied dataset allows the gen AI model to understand different customer preferences, enabling the system to provide personalized and contextually relevant product recommendations.
3. Prioritize data quality
Quality triumphs over quantity when it comes to data foundation for AI. Use tools such as data profiling, cleansing, validation, and monitoring to refine your data, steering clear of inaccuracies and biases that could compromise AI insights.
For example, a provider of healthcare-oriented software may employ data profiling and cleansing tools to make sure that patient records are accurate and error-free. This commitment to data quality enhances the reliability of the AI model's diagnostic insights.
4. Integrate data sources
Integrate data from various sources and formats to create a unified view. This improves accessibility, enriches data attributes, and minimizes silos and inconsistencies. Techniques like data extraction, transformation, loading, and synchronization aid in seamlessly integrating diverse datasets.
For instance, an enterprise resource planning (ERP) software provider can integrate data from various departments into a unified view. This would allow their generative AI models to access and analyze a comprehensive dataset, enriching data attributes and reducing inconsistencies across financial, inventory, and customer management systems.
5. Label your data
Labeling data involves adding annotations or tags to make it understandable for AI algorithms. This step is crucial for training and evaluating AI models, refining data features, and optimizing performance. Techniques such as data annotation, classification, segmentation, and verification facilitate effective data labeling.
For example, to create an AI-based marketing automation tool, you need to label customer data with relevant tags, such as purchasing behavior, preferred channels, and response to promotions. This labeled dataset aids the generative AI model in creating targeted and effective marketing campaigns, ensuring that promotional content resonates with specific customer segments.
6. Augment your data
Augmenting data involves creating new or modified data from existing sources to enhance quantity, diversity, and quality. Overcoming data scarcity, reducing bias, and improving generalization are key benefits. Techniques like data generation, manipulation, transformation, and synthesis contribute to effective data augmentation.
Imagine a financial institution that is eager to use AI-driven software to augment its transaction data by creating synthetic data points. This augmentation technique would help them overcome data scarcity and improve the generative AI model's ability to detect unusual patterns or potential fraud, ultimately leading to improved security and reliability of financial transactions.
7. Secure your data
Establish stringent data security measures to safeguard sensitive information. Implement encryption, access controls, anonymization, consent management, regular audits, and other security protocols to protect your data from unauthorized access, ensuring the confidentiality and integrity of your information.
Suppose, a technology company implements robust data security measures for its generative AI project. This way, the organization safeguards customer data by employing encryption, access controls, and regular audits. This commitment to data security ensures compliance with privacy regulations and builds customer trust.
8. Establish data governance
Formulate and implement policies, processes, roles, and metrics to manage data throughout its lifecycle. Data governance aligns data quality, integration, labeling, augmentation, and privacy with AI objectives, standards, and best practices. Use frameworks and platforms like data strategy, stewardship, catalog, and lineage to establish effective data governance.
For example, for an AI-driven product in the insurance field, establishing data governance policies is crucial for managing customer data throughout its lifecycle. This governance framework aligns with the organization's AI objectives, ensuring that data quality, integration, labeling, and privacy practices adhere to industry standards and best practices.
9. Regularly update your dataset
The business landscape is dynamic, and so should be your dataset. Constantly update your data to incorporate new information and adapt to evolving business needs and trends. This will guarantee your generative AI model remains relevant and effective over time.
For a finance software provider, for instance, regularly updating market data for their AI-driven investment tools will let them have generative AI models that provide relevant and effective insights for investors.
10. Handle missing data
Implement strategies to address missing data, such as calculating replacement values based on statistical methods or deleting records with missing values. These approaches will result in the reliability of your dataset.
A telecommunications company, for example, can address missing data in customer profiles by calculating replacement values based on statistical methods. This approach can help to ensure that customer data for predictive analytics remains comprehensive and reliable, supporting accurate decision-making.
How Flyaps can help you form a solid data foundation for AI
At Flyaps, we have over 10 years of experience bringing business ideas to life with our tech expertise. Here’s our approach to preparing a data foundation for AI implementation.
- We begin by conducting a comprehensive analysis of your data needs, identifying potential challenges, and formulating a tailored strategy. With a keen eye on data quality, we employ advanced techniques for profiling, cleansing, and validation, ensuring that your dataset is accurate and reliable.
- Our team incorporates DevOps practices to enhance the efficiency of the development process. Continuous integration, deployment, and automation ensure a seamless workflow, allowing for quick adjustments based on evolving data requirements. This agility is crucial for adapting to the dynamic nature of AI projects.
- The inclusion of our data engineering specialists further strengthens the data foundation. Our experts design and implement robust data architectures, integrating diverse sources and optimizing data pipelines. Their focus on security and scalability ensures that the data infrastructure can support the unique demands of AI algorithms.
Want to create a well-organized, high-quality data foundation? Our team of skilled DevOps and data engineering specialists will build a strong data foundation specifically designed for your AI needs. Contact us to kickstart the process!