How Much Does AI Cost? Exploring Pricing Factors and Implementation Types
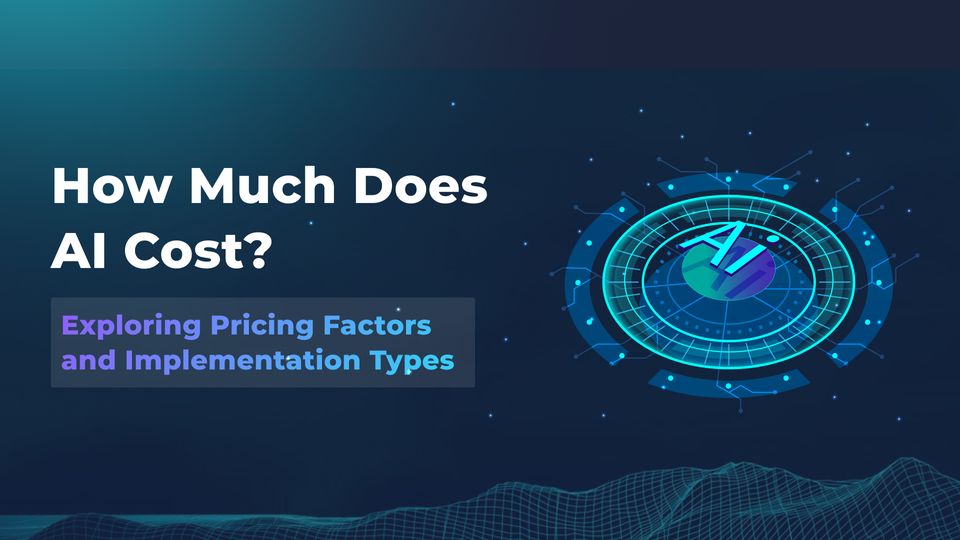
AI has become more common and accessible than ever, with its global market size predicted to increase by 37% each year from now to 2030. If you’re looking to add AI to your project, you probably know there are different options to build AI and the price will depend on which one a specific company needs. AI software solutions range from pre-built solutions offered by third-party providers to custom platforms developed by engineers, each carrying its own price tag.
For custom AI solutions, the costs can vary from thousands to hundreds of thousands of dollars, depending on how complicated the project is and what resources are needed. On the other hand, for third-party AI software like ready-made chatbots, the prices can also differ: you might pay up to $40,000 a year, or get it for free.
In this article, we will answer how much AI costs and shed light not only on price ranges but also on the key factors that influence financial investment across different types of AI solutions. Drawing from our experience of working on projects like Glossary Tech and CV Compiler, our AI-focused software development team is ready to provide valuable insights and real-world use cases of AI implementation.
The typical price range for AI solutions
Let's dive straight into the numbers and talk about the typical price range for AI solutions. Clutch.co, an online platform for getting valuable feedback about IT companies, examined AI development projects on their platform and revealed that those projects typically fall into several cost brackets:
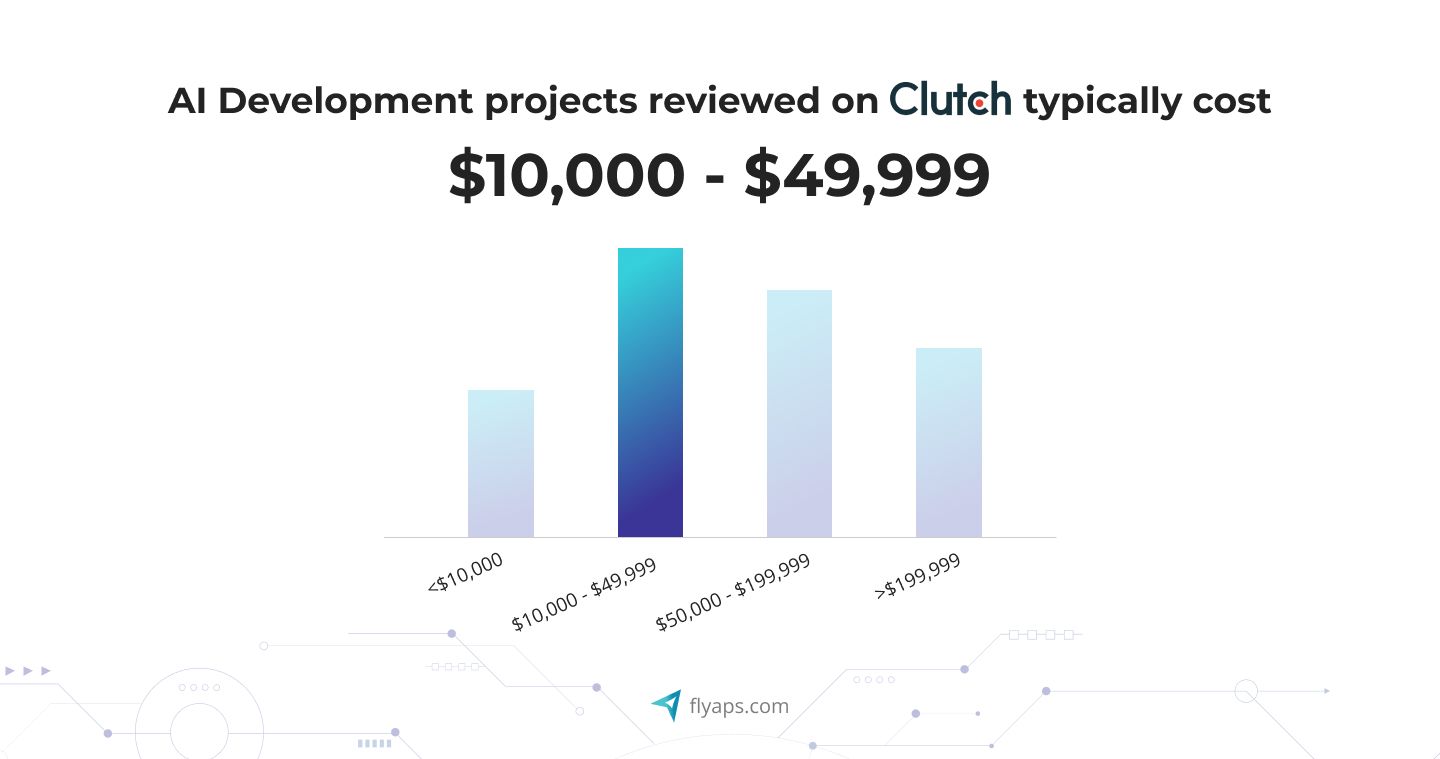
Custom AI solutions in 2024 can range in cost from $6,000 to over $300,000, covering both development and implementation.
Consulting typically comes at a lower cost and varies based on the consultant's hourly rate, which usually falls between $200 and $350. For third-party AI software, like a virtual assistant (which in some cases can even be free), annual expenses could reach up to $40,000.
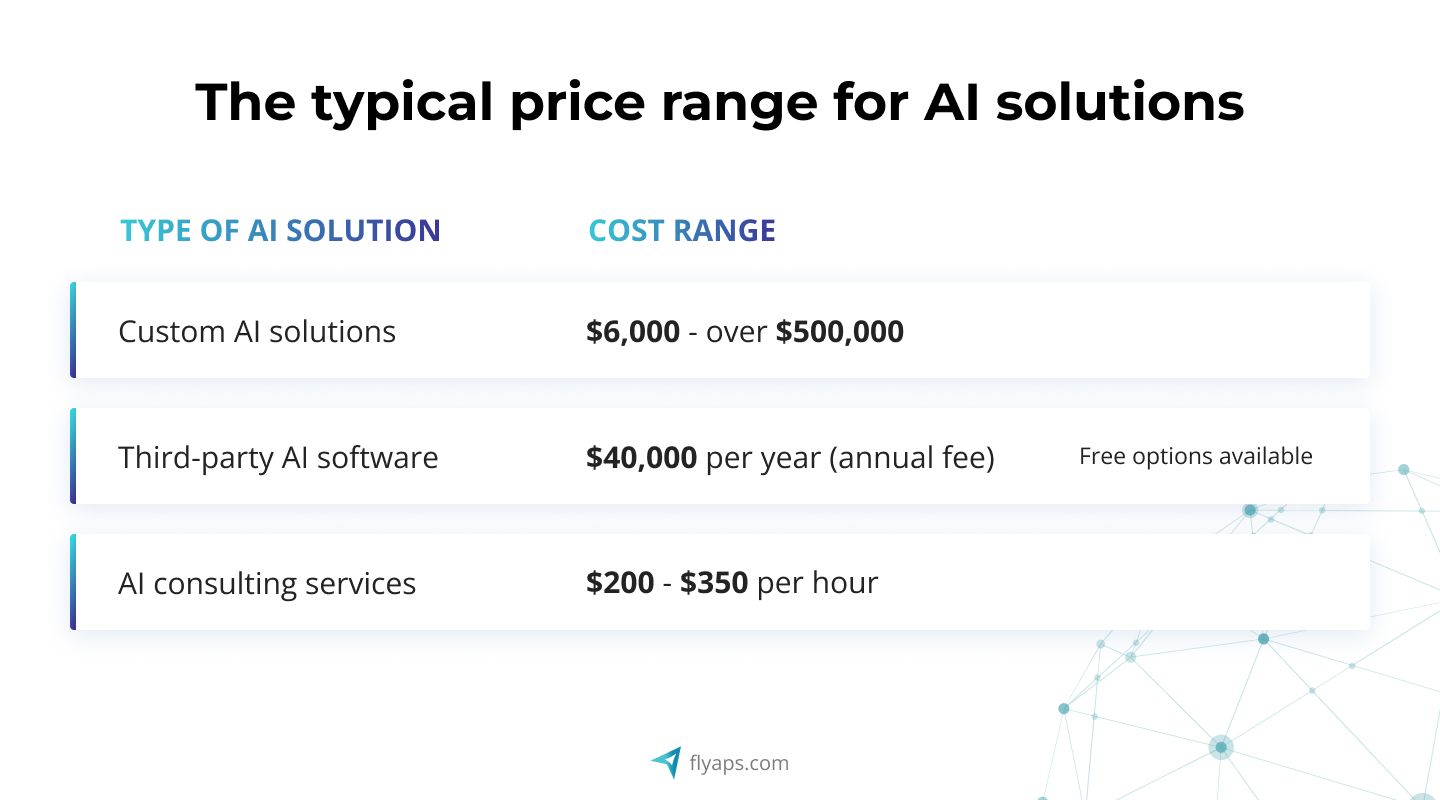
Such wide price ranges are influenced by several factors, each contributing to the final cost of AI solutions.
What determines the AI cost?
The key to keeping any project, including AI projects, within budget is to balance scope, cost, and time (so-called project management triangle). Particularly concerning AI, businesses must also consider four variable factors that can impact the cost of an AI project: the type of AI, project complexity, dataset size, and algorithm accuracy and fluency. Let's take a closer look at each of these variables and how they relate to the project management triangle to better understand their impact on the final project cost.
Type of AI
Different AI applications have varying complexity and performance needs, leading to different scopes of projects and, consequently, different costs. For example, building a basic rule-based system might have a smaller scope compared to developing a deep learning neural network. The complexity of the AI type affects both the time required for development and the expertise needed, thus impacting costs. Here's how it works:
First, think about the basic functions you need and whether you can find that set of functions in ready-made options or if you’re ready to invest in creating your own solution. That is important because AI solutions evolve from being basic and general to becoming highly specialized and intricate over time, regardless of whether these changes align with the specific needs of the customer.
Ready-made options
Ready-made solutions, like chatbots, writing software, recommendation engines, fraud detection and predictive maintenance systems are generally cheaper. They're already built and need less tweaking to work for you.
Let's consider a scenario where a retail company is looking to enhance customer engagement through an AI-powered chatbot on their e-commerce platform. Opting for a pre-built solution, developers integrate an off-the-shelf chatbot equipped with standard conversational capabilities and basic product recommendation algorithms. Here’s an approximate cost breakdown:
1. Software license
Let’s say the company decided to go with a plan with high customization options for businesses. Such subscriptions cost from $600 to $5,000/mo. The price includes acquiring the pre-built chatbot software license, covering initial setup and access to extended features.
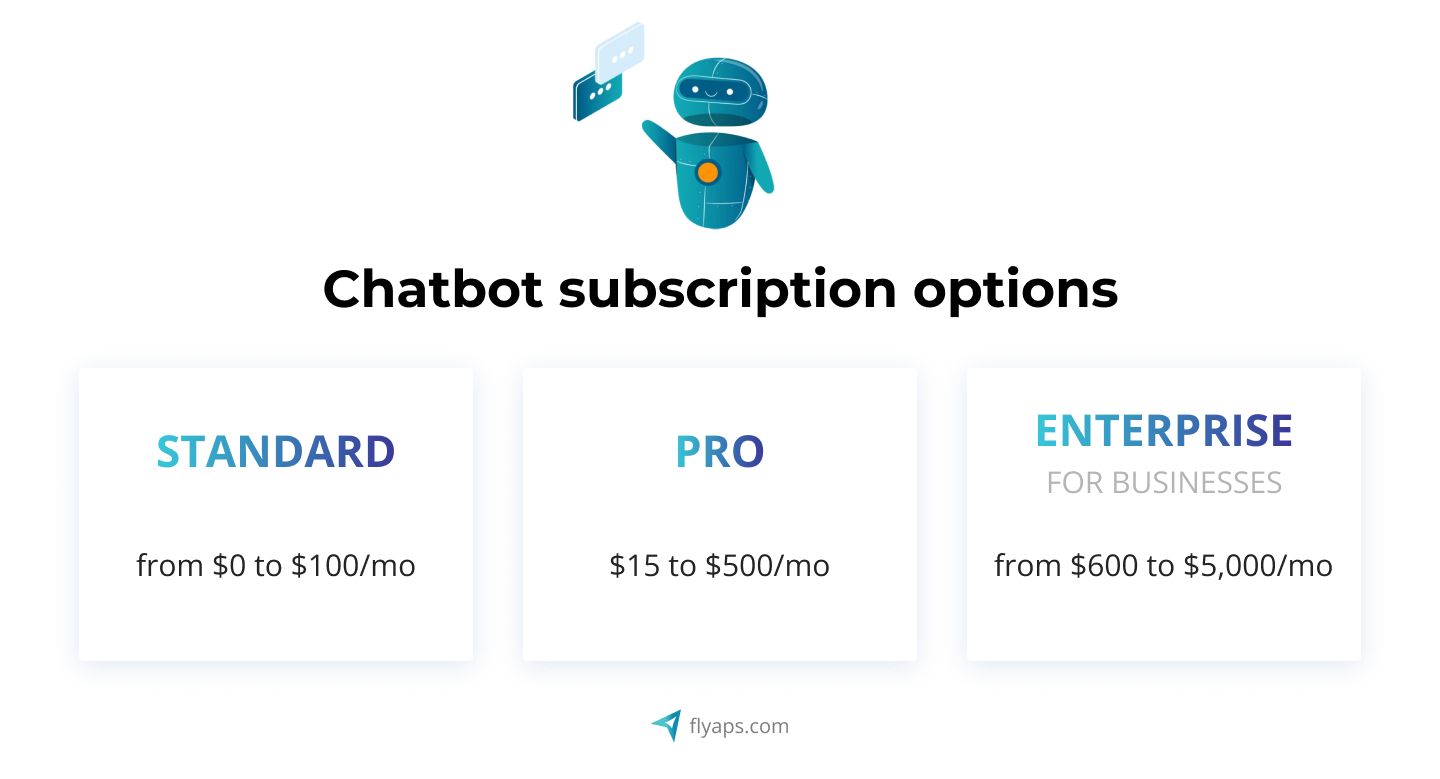
ChatBot, for example, offers such options:
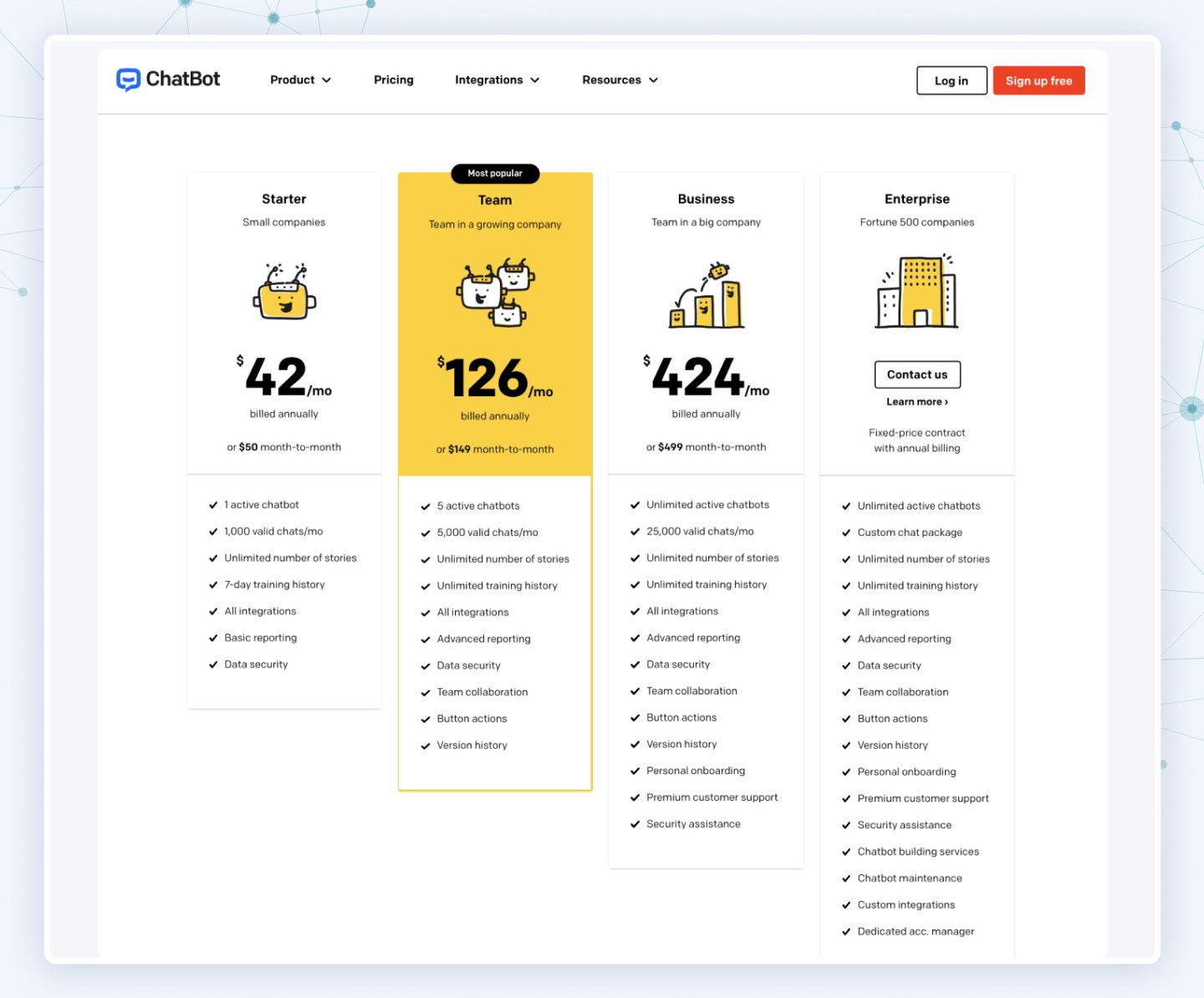
2. Integration and customization
Though the enterprise plan provides some customization, the company needs developers to integrate the solution into the company’s ecosystem and customize its appearance to align with the brand identity. The cost range for this stage depends on the salary rate of tech specialists. Let’s assume, the company paid at least $4500 for all the work.
3. Training and support
Ongoing support and training expenses ensure seamless operation and troubleshooting as the company's customer base grows and usage patterns evolve. Suppose, the bar for this stage is $2,000.
To sum up, let’s compare the most significant pros and cons of off-the-shelf AI software in terms of price impact:
Custom AI development
Custom solutions, like supply chain optimization tools, analysis systems, process optimization, and virtual assistants, tend to cost more than off-the-shelf software. However, such solutions are tailored specifically to your needs, which will address your business challenges more efficiently in the long run.
Let's envision a scenario where a tech startup embarks on developing a custom AI-driven recommendation engine for personalized content delivery in a news aggregation app. The cost breakdown, in this case, will look like this:
1. Requirement analysis and design - at least $3,900
Extensive consultation and requirement analysis sessions are conducted to conceptualize the tailored recommendation engine's functionalities and user interface design.
2. Development and testing - minimum $13900
Skilled AI-focused software developers with a proven track record of successful AI and ML cases start an iterative development process, coding and fine-tuning complex algorithms for content recommendation, ensuring accuracy and relevance. Rigorous testing phases are crucial to guarantee robust performance across diverse user scenarios.
Furthermore, to optimize the performance of an AI model and ensure it delivers the best results, additional training is often necessary. This additional training involves iterating on the model, fine-tuning parameters, and potentially incorporating new data to enhance its accuracy and effectiveness. The practical implementation of additional training involves considerations beyond just the algorithms and data but also the infrastructure required. The infrastructure encompasses the resources, such as GPUs or TPUs, that are essential for handling the computational demands of training large-scale models and processing vast datasets.
The infrastructure cost associated with additional training can significantly impact the bottom line of a project. It's not uncommon for these expenses to range from hundreds to a few thousand dollars.
3. Deployment and maintenance - around $400 per month
Post-deployment, ongoing maintenance and optimization efforts are essential to address any performance issues, adapt to evolving user preferences, and incorporate feedback-driven improvements.
The total cost range for a custom solution, in this particular case, will be between $20,000 and $30,000.
With that being said, let's delve into the major pros and cons of pre-built AI software from a cost perspective:
Project complexity
More complex systems generally cost more to develop and maintain, even though they typically evolve from simpler solutions with basic functionalities gradually growing to complex ones. For better understanding, let's compare building a chatbot for customer interactions with developing an AI system for language and image recognition, or an analysis system.
Imagine an e-commerce platform looking to enhance its customer support services. They decide to implement a chatbot to handle customer inquiries, streamline support processes, and improve user experience.
Building a chatbot typically involves integrating existing natural language processing (NLP) models and frameworks like Google's Dialogflow or Microsoft's Bot Framework. These tools often come with pre-built functionalities for understanding and responding to user input, which can significantly reduce development time and costs. Additionally, deploying a chatbot usually involves setting up a backend infrastructure to handle user requests and integrate with other systems like customer databases or ticketing systems.
Google Dialogflow offers a free standard plan for small and medium-sized businesses, and the Enterprise edition, which costs $0.0002 per request. Microsoft's Bot Framework is free for up to 10,000 messages per month. After this limit is exceeded, a paid plan is available starting from $0.50 per 1,000 messages.
Now, let’s consider a scenario where a startup developing medical imaging software for diagnosing diseases from scans and images. They require advanced AI systems for language and image recognition, as well as robust analysis capabilities. Such a project would involve:
- Data collection and labeling large datasets for training AI models, which can be costly. It may involve purchasing proprietary datasets, hiring data annotators, or investing in tools for data labeling automation. Additionally, ensuring data quality and diversity adds to the expense, as it may require extensive validation and verification processes.
- Training AI models on powerful hardware, such as GPUs or cloud-based platforms. Cloud-based ML platforms often charge based on usage, including training time and resources consumed. Fine-tuning and optimizing models may also require iterative experimentation, which prolongs the training process and increases costs.
- Setting up scalable and reliable infrastructure for deploying AI systems, which involves both initial setup costs and ongoing operational expenses. Cloud-based deployment options offer flexibility but come with usage-based pricing models, meaning costs can scale with the number of users or inference requests. Implementing features like load balancing and fault tolerance adds complexity and may require additional investment in infrastructure.
Add to this list the ongoing monitoring and maintenance expenses of AI systems, and it will be even clearer how the cost of the final product grows with each stage of AI development.
The size of the data set
AI's effectiveness depends on the data it's trained on, as data is crucial for the quality of the model. More and better data usually means higher costs. However, open-source datasets like Google Cloud Public Datasets, Kaggle or Registry of Open Data on AWS can help mitigate expenses related to data acquisition.
The availability of pre-trained AI tools like large language models (LLMs) can simplify the training process. Such tools as ChatGPT or DALL-E 3 are often ready to use without customization. However, for the best results, it's ideal to fine-tune these algorithms using data related to your company.
Different AI models come at varying costs, and one crucial factor is the size of the context window. The context window refers to the amount of background information the user provides to the AI.
Let’s say you want an AI to predict stock market trends based on historical data. Giving it only a few days' stock prices won't be very effective. However, providing years' worth of diverse market data, including economic indicators, company performance metrics, and industry news, can help the AI learn patterns and make more accurate predictions.
The same goes for pieces of text. Tomasz Tunguz, an author of data-focused blogs, emphasizes that a 500-word essay costs about 8.4 cents when created with the most advanced version of the GPT-4 model. However, using a less sophisticated model like Llama2, an open-source model from Facebook, the cost drops significantly to just 0.07 cents. The difference in cost between these top-tier and free AI models is huge — about 120 times cheaper to use the free version. However, these prices can change. OpenAI recently announced a 3x reduction in costs, and this trend of reducing costs is likely to continue as engineers improve the technology behind AI.
Algorithm accuracy and fluency
Accuracy and fluency of AI solutions are particularly important when it comes to generative AI. AI can be accurate in predictions for important business decisions or highly fluent to sound natural and human-like. Even businesses without tight budgets have to choose one thing over another because the more fluently AI “speaks”, the less accurate it can be.
For example, a customer support chatbot is usually expected to handle up to around 60% of routine tasks and simple user queries, so it needs to be highly fluent. For complex issues, there’s always a human specialist waiting on the other end of the line and it can use different types of AI to perform calculations, forecasting, or some automation processes, which are expected to be highly accurate.
Achieving higher accuracy often requires more sophisticated algorithms, extensive testing, and fine-tuning of models. These processes demand additional resources, including specialized expertise, computational power, and time.
Furthermore, maintaining high accuracy levels over time adds to the project's cost. AI algorithms need continuous learning and updates to adapt to changing environments and improve performance, which incurs ongoing expenses for training and maintenance.
Conversely, developers may prioritize efficiency over precision if the AI's tasks are less critical or do not require great accuracy. This approach can reduce costs by simplifying algorithms and reducing the need for extensive testing and optimization.
How Flyaps can help you with AI implementation
With over a decade of experience helping businesses worldwide adopt trending technologies and improve their operations and offerings, our team has extensive expertise in AI solutions. We can help you implement the best AI options suited to your budget.
Here's what we offer:
Integration of third-party AI (including generative AI)
Through Flyaps, you can seamlessly integrate third-party AI solutions (including large language models (LLMs) some generative AI tools are built on), into your existing infrastructure. This approach allows you to leverage the latest advancements in AI technology without the need for extensive development efforts.
Ready-made AI solutions built by Flyaps
Flyaps provides ready-made AI solutions, like those we've introduced in a previous section, that empower you to build AI capabilities significantly faster. These pre-built frameworks are meticulously crafted to address specific industry challenges and tailored to your unique requirements.
Custom AI development
When building custom AI applications from scratch, our team always starts with a Proof of Concept (PoC) to avoid wasting resources. Our Agile approach enables you to prove the feasibility of your AI project through iterative development cycles, minimizing risks and maximizing efficiency.
Still not sure how to calculate the final cost of your AI-driven project? Just drop us a line and we will assist you every step of the way.