6 Steps for Generative AI Product Development
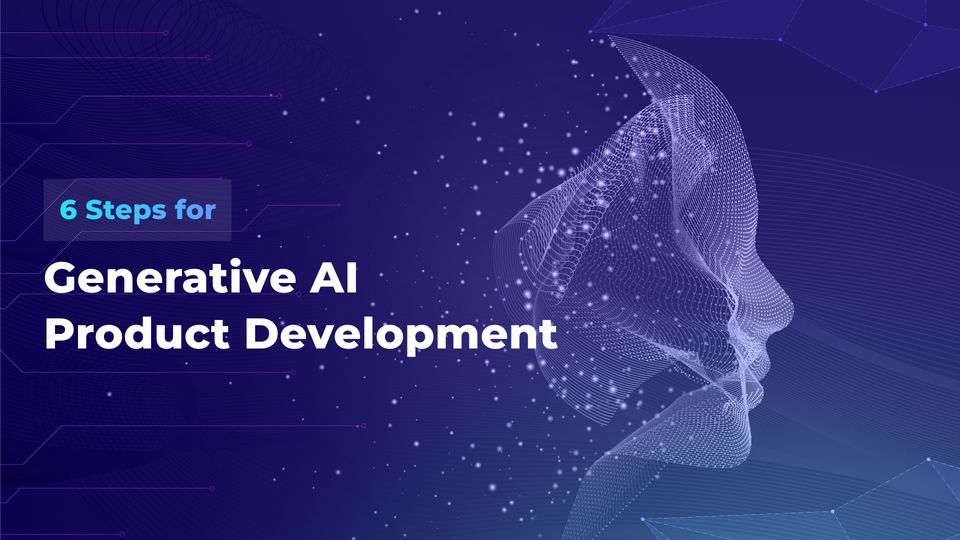
The world of generative AI is booming, going from a $40 billion industry in 2022 to an expected $1.3 trillion in the next decade, growing at a rate of 42% each year, according to Bloomberg. As more and more companies enter the market every year, it’s already getting a bit crowded there.
Such a surge in competition makes it tough for companies to stand out. Even big companies seem to rush in without really checking if their clients actually need a gen AI product. Take, for example, Microsoft's recent attempt to integrate AI into Windows, which was met with little enthusiasm and even faced backlash on Reddit and was labeled as unnecessary.
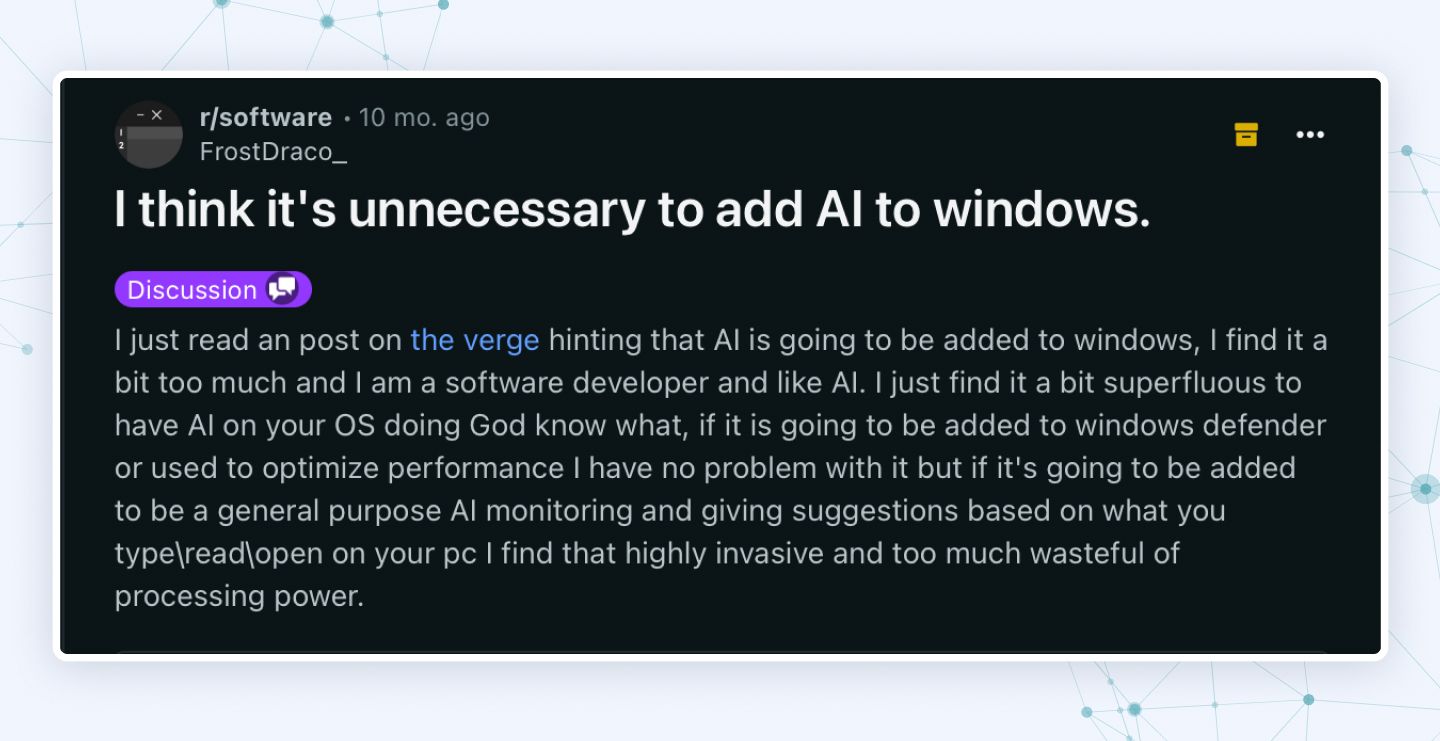
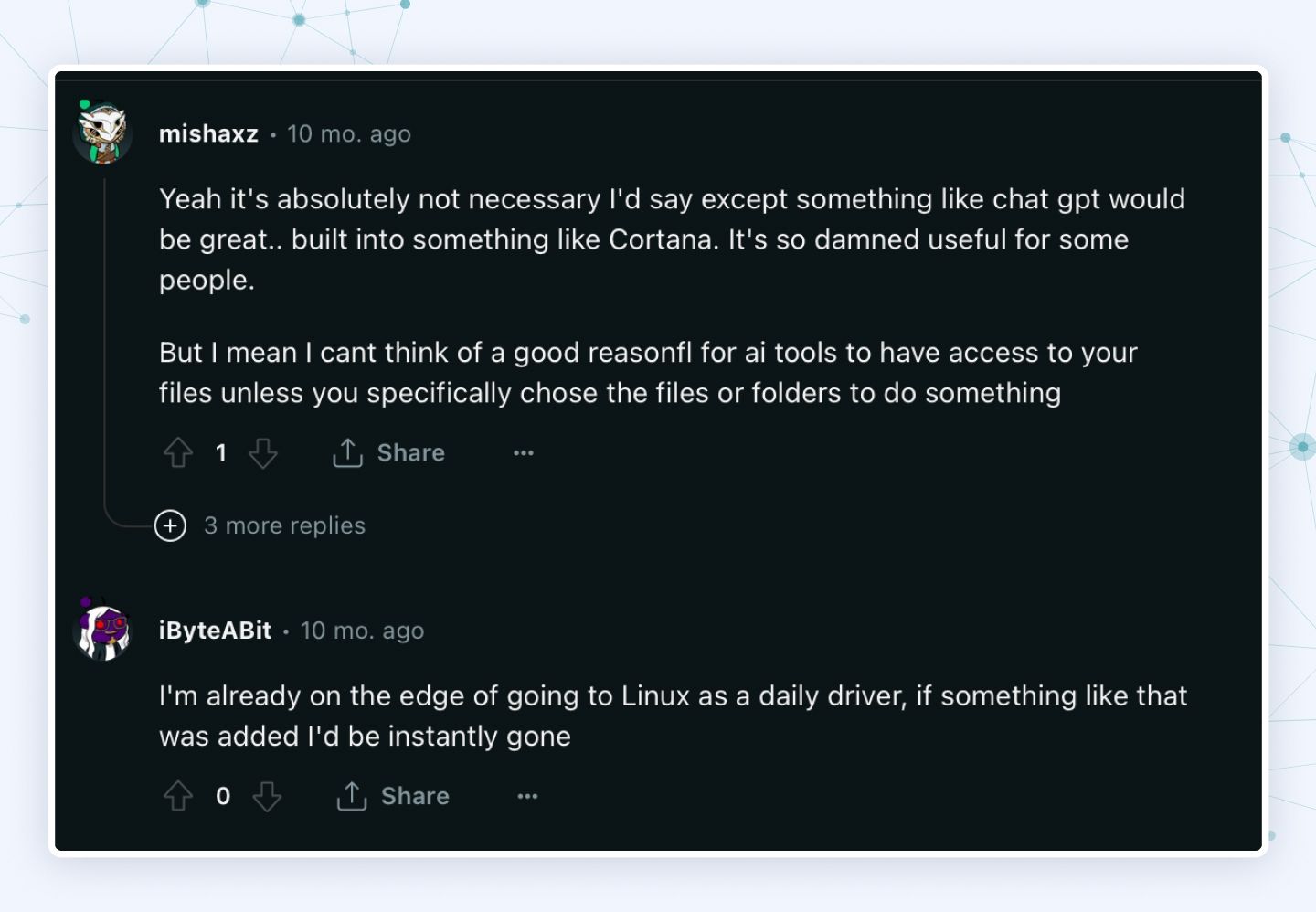
At Flyaps, we've been in the AI game for over a decade, helping companies grow with our tech assistance and innovative solutions. To help you navigate the generative AI market and avoid common pitfalls like choosing the right gen AI feature that would be in high demand, we’ve put together a comprehensive guide. Follow these 6 steps of generative AI product development to thrive in this rapidly evolving landscape.
Step 1. Focus on a customer-centric approach
In such a fast-changing environment, staying relevant to users is the Holy Grail. Therefore, generative AI product development begins with a profound understanding of not only the current pain points faced by clients but also the foresight to anticipate how these needs might transform in the future.
Imagine a forward-thinking company crafting innovative and smart solutions for banks and other financial organizations. Let's call them FinTechSolutions. With a track record of providing cutting-edge technology to enhance operational efficiency, they now stand at the threshold of developing a groundbreaking generative AI product for their clients.
Let’s look at four strategic questions guiding FinTechSolutions to determine the challenges their clients face:
1. What specific problem are our clients facing in their day-to-day operations?
FinTechSolutions starts by identifying the specific challenges faced by banks and financial organizations. Whether it's streamlining complex processes, improving data security, or enhancing customer experiences, pinpointing users’ pain points is the crucial first step.
2. How common is this issue among our client base?
Understanding the scale of the issue is paramount. FinTechSolutions conducts thorough market research to determine the number of financial institutions grappling with the identified pain points. This step ensures that their generative AI product would have a widespread impact.
3. Is it urgent enough to take action?
Beyond mere identification, FinTechSolutions evaluates the severity of the pain points. They prioritize challenges impacting operational efficiency, customer satisfaction, or overall performance. The focus is on addressing issues that demand extra attention and innovative solutions.
4. Are non-AI tools effectively tackling the issue, or is there a gap that requires a more advanced approach?
Before diving into generative AI product development, FinTechSolutions assesses existing tools and solutions in the market. They seek to understand whether conventional technologies adequately address the identified pain points. If non-AI tools can’t solve the issue effectively, it becomes a prime candidate for a generative AI solution.
After deciding on key pain points and features that can solve them, it’s time to move on to the next step, which is to work on either system accuracy or fluency.
Step 2. Choosing between accuracy and fluency
When possibilities of using generative AI range from performing abstract poems to advising for making critical business decisions, choosing an expected level of accuracy of the product is a second crucial decision after determining pain points. The same goes for fluency. By fluency, we mean the ability of the generative AI products to sound natural and human-like. Take ChatGPT, for example. People notice how smoothly it talks more than how accurate it is. This affects how we use the system - it's great for making interesting content, but we are watchful and highly critical when it comes to ChatGPT's pieces of advice on topics like tech or finance. So, deciding on the right balance of accuracy and smoothness is key to making generative AI work well for different tasks.
Coming back to our earlier-mentioned FinTechSolutions, they are looking for ways to solve this dilemma between accuracy and fluency by finding answers to the following questions.
Need for accuracy:
- How crucial is precision for the specific use case?
- Is pinpoint accuracy essential, or can the margin of error be flexible?
Need for fluency:
- Is a fluent, natural narrative crucial for the intended purpose?
- Does the need for fluent communication align with the practicality of the task at hand, such as providing data for business decisions?
The thing with large language models (a core of generative AI) trained for dialogue is that they are programmed to give users an answer no matter what. If they don't know how to answer, they just make it up and sound pretty confident and fluent in what they're saying. To be more specific and accurate such models have to be trained on huge amounts of data.
Let’s imagine that our FinTechSolutions found out that the biggest pain point for their clients is a decrease in customer satisfaction resulting from a delay in answering calls and letters. The company decided to create a chatbot, models of which are taught to provide simple answers to the most asked questions, as well as provide basic consulting services.
Is accuracy essential for financial consulting? Definitely. Does the provided info need to be in clear and simple language? Indubitably. Does that mean that the company must choose and compromise on accuracy or fluency? In our case, it depends on the budget of the project and the amount of data the company can provide for models to learn from. “Use cases related to improving creator/workplace productivity are less complex/risky and could be a better fit for current LLM/generative AI technology vs. information-seeking/decision-support use cases,” said Barak Turovsky, VP of AI at Cisco and former Head of Languages AI product teams at Google.
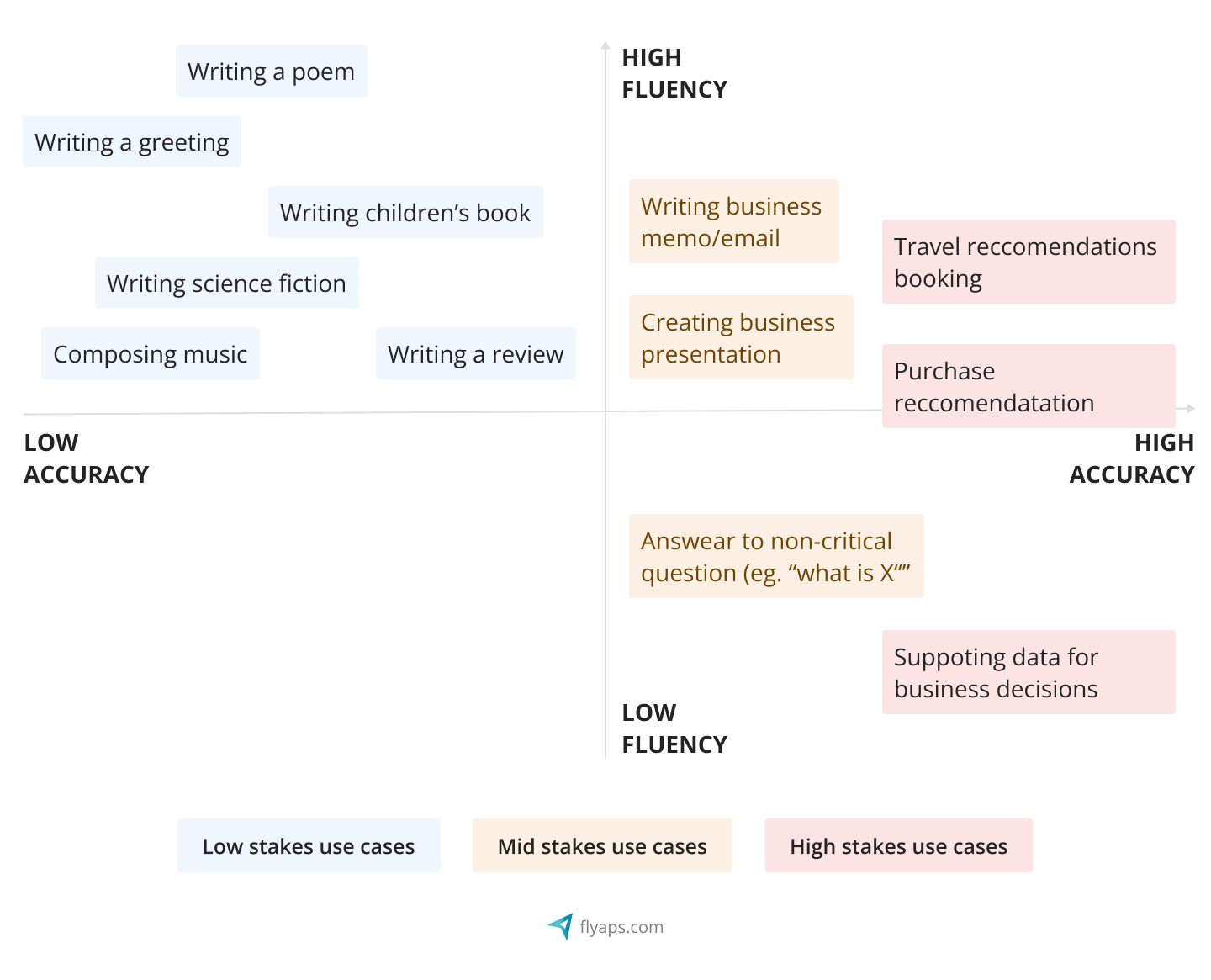
Step 3. Challenge established competitors
The current market for generative AI is already filled with tools that can handle basic tasks. For new players to make a mark, they need to stand out by being innovative and creative. Newcomers now have at least five approaches to challenge other players on the market. Let’s break each of them down.
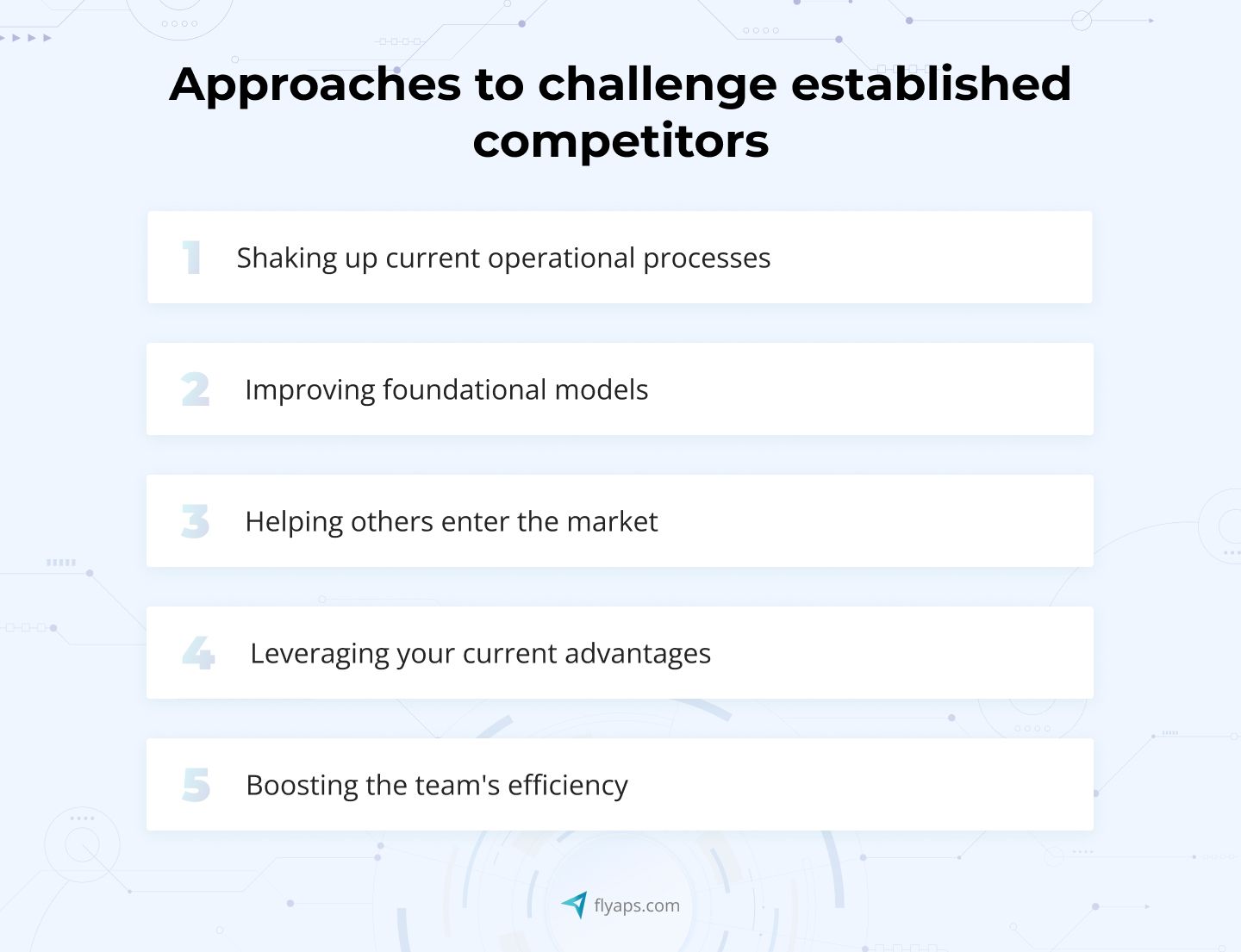
Shaking up current operational processes
Adding gen AI to existing products is the fastest way to enter the market. But, instead of doing this, you can completely redesign them to take full advantage of a generative AI product.
By changing current systems this much you can make established competitors lose their advantages in terms of data and distribution. This is especially true for tasks that were traditionally done manually. For our imaginary company working in the fintech domain, for example, they can create a system for automating contract creation between financial organizations.
Overall, it's about using AI to revolutionize how products and services are built and delivered, potentially making existing ones obsolete.
Improving foundational models
Startups can make their mark by making foundational models, like GPT-3.5, even more helpful, and then monetizing this improvement. They can use prompt engineering - a method to train language models to give desired outputs by understanding the instructions. With output chaining, models would be able to connect the output of one system or device to the input of another. It's like linking different components together in a chain so that the output of one part becomes the input for the next. Improving models’ user experience and interface - another area to enhance.
Helping others enter the market
AI-models-as-a-service providers are becoming popular among enterprises looking to build their own gen AI models. Companies like Mistral, Stability AI, and Sana are successful in working in this field.
Other opportunities for startups include supporting companies in organizing and getting their data ready for gen AI models, training these models, and evaluating, experimenting, and putting safety measures in place.
Leveraging your current advantages
For startups that have already started and are searching for the next big leap in growth, combining generative AI with existing strengths like human expertise, a solid customer base, or excellent distribution channels can be a game-changer. Using gen AI, for example, to enhance user experience can set off a positive cycle: a better user experience can attract more users, generating more data that can then be used to improve the foundational model and make the experience even better.
Boosting the team's efficiency
A generative AI product is likely to bring a significant benefit in terms of increased productivity through automation and assisting human labor. On the external side, companies can use gen AI to cut costs in areas like sales, marketing, customer service, and content creation. Internally, the same applies to tasks like bookkeeping, creating presentations, summarizing meetings, data analysis, coding, and much more.
Step 4. Figure out your moat (competitive advantage)
Moat is a business's ability to maintain a competitive advantage, safeguarding its long-term profits and market share. Here are some key considerations for startups to build a robust economic moat when developing a generative AI product strategy:
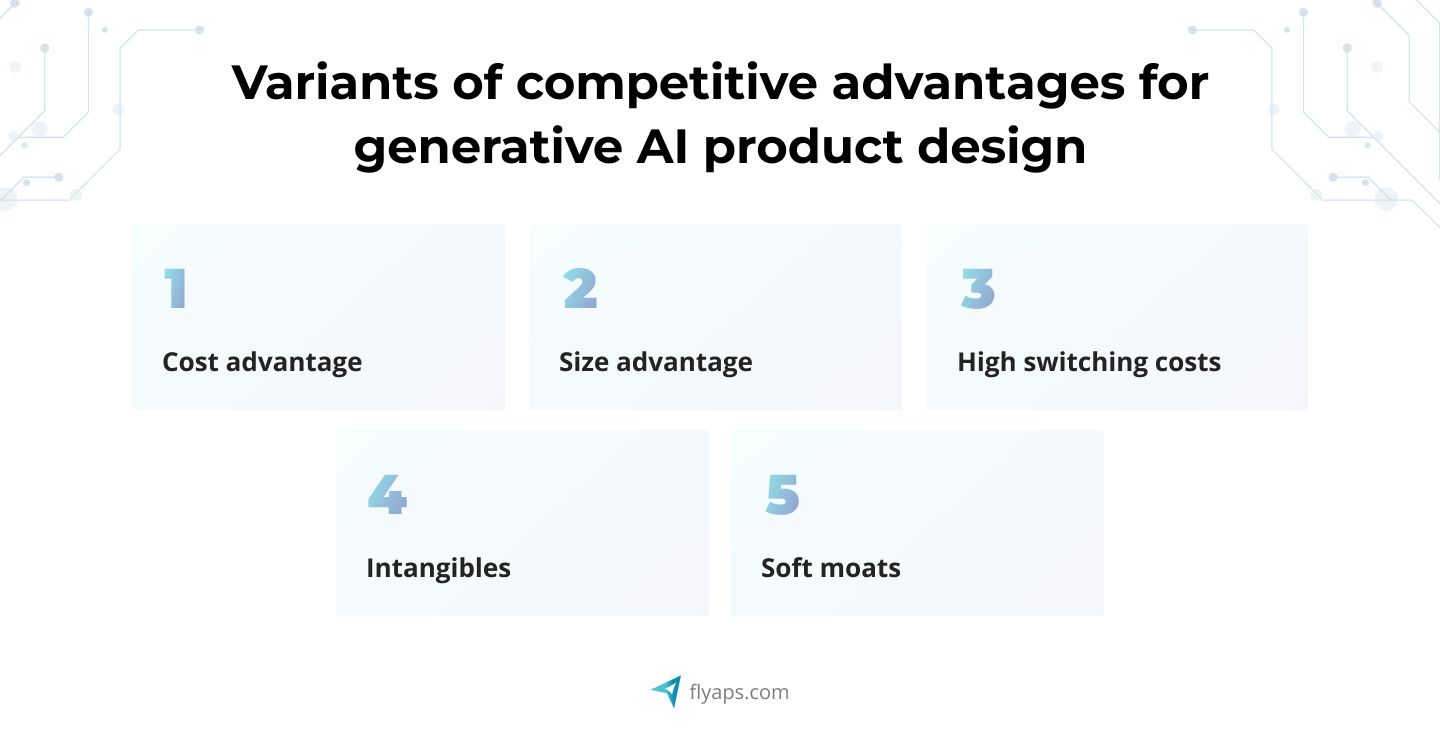
- Cost advantage
Achieving a cost advantage that competitors can't easily replicate becomes a potent economic moat.
In the case of FinTechSolutions, which we've mentioned before, by developing highly efficient and automated backend processes, the company can achieve a cost advantage in transaction processing. This lets them offer lower fees than competitors, making it challenging for new entrants to match their pricing structure.
- Size advantage
Size matters. Larger companies often benefit from economies of scale, reducing costs and dominating the core market share. This can create a barrier for smaller competitors, confining them to niche roles.
Suppose, FinTechSolutions grows and now the company can negotiate better rates with financial institutions and benefit from economies of scale in processing financial transactions. This size advantage allows them to provide a comprehensive suite of services at a lower cost compared to smaller competitors.
- High switching costs
Once established, a company can impose high switching costs on suppliers and customers.
Once businesses and consumers integrate FinTechSolutions's tools into their financial workflows, the costs associated with switching to a competitor become substantial. The intricacies of migrating financial data and adapting to a new system create a significant barrier, contributing to FinTechSolutions' market dominance.
- Intangibles
Intangible assets, like patents and brand recognition, form another type of economic moat. Strong brand recognition allows companies to charge premium prices, boosting profits.
For instance, FinTechSolutions can establish a strong brand through its commitment to data security and user-friendly interfaces. The trust built around their brand recognition allows them to charge extra for their services, attracting customers who prioritize reliability and innovation in financial technology.
- Soft moats
Exceptional management or a unique corporate culture can create “soft moats,” contributing to a company's prolonged success. Although challenging to quantify, these factors play a visible role in establishing a lasting competitive advantage.
For example, if the leadership team at FinTechSolutions fosters a unique corporate culture that promotes innovation and customer-centricity, the intangible quality contributes to their prolonged success, creating a workplace environment that attracts top talent and inspires ongoing advancements in financial technology.
Step 5. Realistic growth assessment
Unlike traditional AI, generative AI is more complex, making it challenging for new and small companies to enter the market due to the associated time, cost, and expertise. However, not everyone will achieve success and revenue growth simply by building generative AI applications. Here are key areas where startups can make an impact:
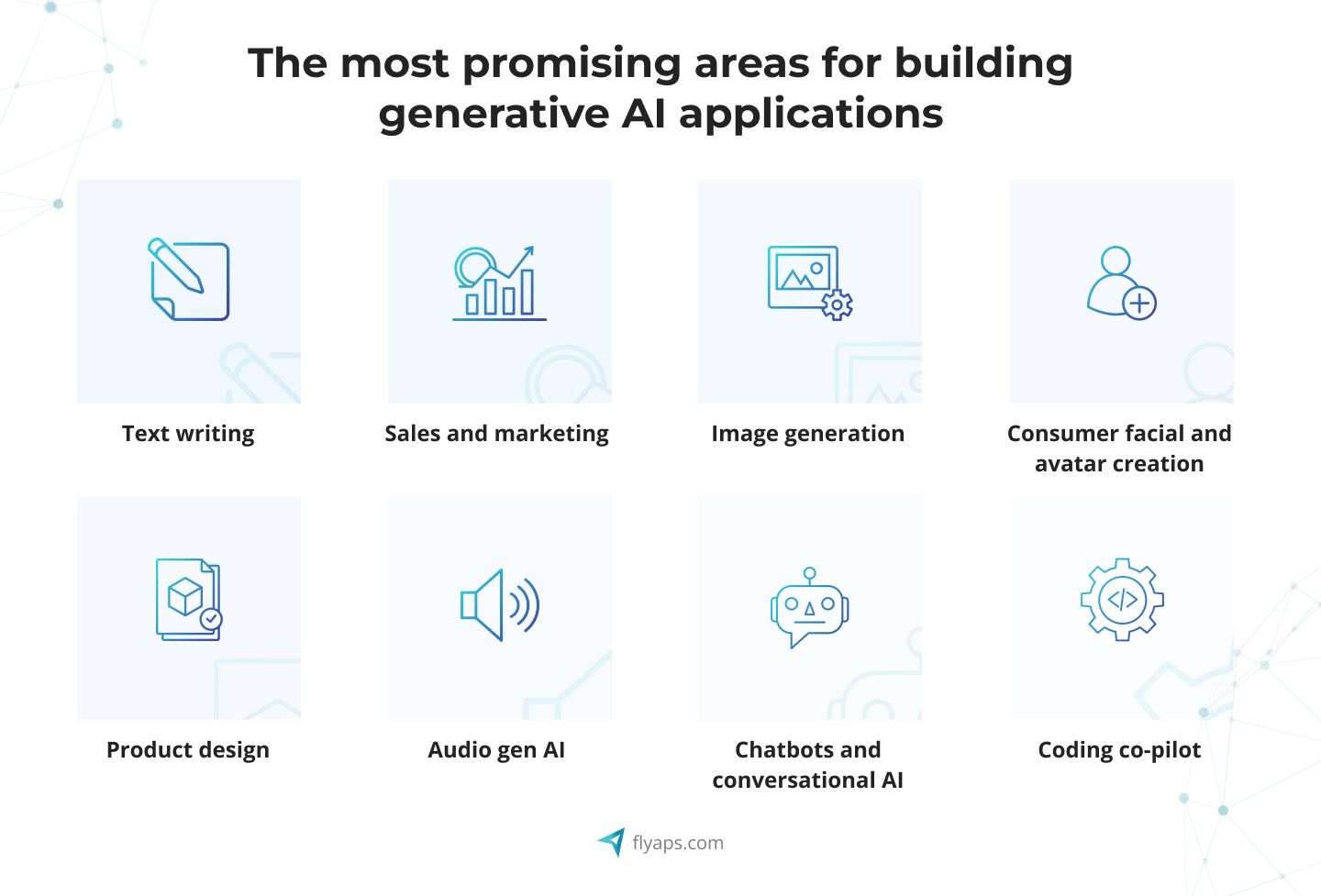
- Text writing
Companies can assist users in real-time with everyday writing tasks, such as email composition, document creation, and text form completion. Some prominent examples are Wordtune and Grammarly.
- Sales and marketing
The creation of high-converting textual content for sales and language generation, like Jasper.ai and Anyword do, is at its peak.
- Image generation
By leveraging generative AI models like Open AI’s DALL-E-2 and Stability AI’s Stable Diffusion startups can create tools like Art Breeder that help users create collages.
- Consumer facial and avatar creation
Apps for creating profile pics for social media, such as Facetune, Lensa AI, and Reface have a lot of potential these days.
- Product design
Innovating in areas like fashion shoots, architectural plans, floor plans, and automation of construction documents, can help small startups succeed. Examples include Botika, Maket, and TestFit.
- Audio gen AI
These include music generation services, voice-over solutions, and speech-to-text services. Startups like Soundraw, Boomy, and Deepgram operate in this space.
- Chatbots and conversational AI
For this category, you can choose to build vertical-specific health symptom checkers and superhuman voice assistants, like Healthily or Cresta AI.
- Coding co-pilot
Build on the success of tools like GitHub Copilot, focusing on automating code generation. Notable startups in this niche include Warp and Tabnine.
Step 6. Find a reliable tech partner
Teaming up with an experienced tech partner is crucial for ensuring your project's success. When looking for a partner, consider businesses with a strong background in both AI and generative AI. Your ideal collaborator should resonate with your vision, grasp your specific needs, and possess the technical know-how to bring your generative AI ideas to life.
Consider Flyaps as an example. Our portfolio includes successful AI projects like the GlossaryTech extension and CV Compiler, and our products are actively used by more than 100 companies worldwide, including giants like Orange Group, Telenor Group and Yaana Technologies. Leveraging our expertise and the trust of renowned companies, we can provide invaluable assistance, from refining algorithms to optimizing performance, playing a pivotal role in the triumph of your generative AI product.
In summary, ask yourself these 5 key questions
To recap all the above, before jumping into generative AI product development consider these five crucial questions.
1. Is the product solving a problem without AI?
Before getting dazzled by the AI buzz, ask if the product genuinely solves a problem. If you take away the AI, does it still serve a purpose?
2. How precise does the solution have to be?
Consider the level of accuracy needed for the solution. Does it require pinpoint precision, or is a more general accuracy acceptable?
3. How quickly will competitors respond?
Think about the speed at which existing companies in the market might come up with similar gen AI products. How fast can they catch up to or surpass the proposed AI solution?
4. Is there a moat?
Assess whether the AI product has a competitive edge. Is there something that protects it from quick replication or competition?
5. Does the valuation make sense?
Finally, critically evaluate the company's valuation. Does the perceived value align with the actual worth and potential of the AI product?
Don’t have all the answers or worry about the tech part of your generative AI development process? We’ve got you covered. Drop us a line, and let us handle all the intricacies.