Open Source vs Closed Source AI: Making the Best Choice for Your Business
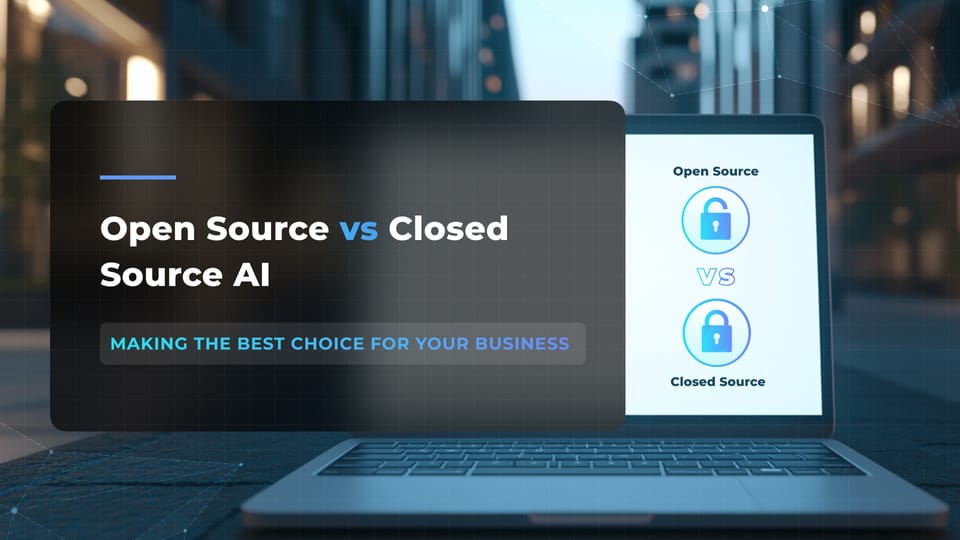
The debates over open source versus closed source artificial intelligence have divided businesses into two distinct parties. One party pushes the open source AI agenda, with transparency, collaboration and cost savings being the key selling points. At the same time, another party advocates for closed source AI due to such benefits as tighter control, enhanced security and better risk management.
Deciding which way to go isn't always easy, especially when transparency, pricing and security are at stake. As an AI-focused software development company, at Flyaps we've dealt with both open and closed AI. That's why we've decided to translate our 10+ years of experience in the market into useful insights on the key differences between open and closed source AI, and the key factors you need to consider when choosing between them.
Open source and closed sourced AI: a simple breakdown
Before we jump into the detailed comparison of these two concepts, let’s kick things off with the basics so that we’re all on the same page.
Open source AI is like a community project — anyone can view, modify, and share the code. It’s all about collaboration and transparency, where developers from around the world can contribute and improve the software. Its openness allows a large community of developers to contribute to its growth and use, which results in high performance and accuracy. Take Hugging Face, for example. It has built its entire business model around open source models, making these powerful tools easy to access and understand for developers and organizations alike.
The source code of closed source (or proprietary) software, on the other hand, is not publicly available. Only the original developers or those with permission can access and modify the code. It’s usually developed in-house by companies that want to control and protect their intellectual property.
In the AI domain, OpenAI’s models (like GPT-4) serve as an example of a closed source. While the technology is widely known and used through various applications, the underlying model and code are not accessible to the public.
So, one is free and offers more customization, and the other comes at a price but offers improved security. Sure thing, this information alone isn’t enough to make an informed decision. That’s why we now move to the main topic of our discussion: the key differences between these concepts that you need to consider when choosing one for your projects.
Key differences between open source AI and closed source AI
There are several aspects that will impact your choice between open source and closed source AI: how each serves your business needs, their complexity for integration, the size of your budget, and, last but not least, their alignment with your security requirements. Let's look at each of these aspects.
Business needs
If your business is in a highly specialized field, like medical diagnostics, pharmaceutical research, or fraud detection, then closed source AI models are usually the winner. They are designed with industry-specific features in mind and often come with access to proprietary datasets that are crucial for accuracy and reliability in tasks where there’s little room for error. For example, in medical diagnostics, closed source AI can analyze detailed patient data and imaging with a level of precision that’s essential for making accurate diagnoses.
But if your needs are more general, like handling customer service, summarizing text, or generating content, open source AI would be a better fit. These models are incredibly versatile and can be adapted for a wide range of tasks without needing any specialized data. For instance, an open source AI could easily be trained to manage customer inquiries or create summaries from long documents. Plus, they’re often more budget-friendly and benefit from continuous improvements thanks to a global community of developers. So, for general everyday tasks, open source AI is often all you need.
Let’s sum up:
Models’ complexity and ease of integration
When talking about AI models, several additional factors need to be considered. They are the depth of the algorithms (how many layers of processing models go through), the amount of data they require, the computational resources needed, and the level of expertise required to deploy and maintain them. That's what we mean by the level of models' complexity, and it can significantly impact how these models fit into your existing infrastructure and operations.
As an example, consider a financial institution using an advanced closed source AI model for fraud detection. They might find that their current database systems struggle to keep up with the real-time data processing the AI needs. These closed source models often come with highly advanced algorithms that need a lot of computational power. In this case, they might have to invest in high-performance databases or even a distributed computing framework like Apache Spark. Plus, since the AI is closed source, they have less flexibility to tweak it to fit the existing setup. Customization may add to the final cost, when in some cases the institution might need more investment in new hardware or software.
On the flip side, open source AI models tend to be more straightforward and flexible, especially if your team has some experience with AI. These models are usually modular, which means you can start with the basics and add more features as needed, making the whole process a lot easier to manage. For instance, if you’re a small business looking to introduce AI-driven customer service, an open source model like TensorFlow or PyTorch could be a great choice. You could start with something simple, like a chatbot, and then build on it as your needs evolve. The flexibility here is a huge advantage — your team can tailor the AI to suit your business as it grows.
So, how do you balance complexity with ease of integration? If your team has the technical chops and you want maximum control, open source AI might be your best bet. But if you need something that integrates quickly and comes with strong support, closed source AI could be the way to go.
Some businesses mix and match, using closed source AI for critical tasks where security and compliance are paramount, and open source AI for more flexible, innovation-driven projects. This way, you get both — powerful features and flexibility.
Cost implications
We have already touched on the issue of costs, but let's discuss this topic in more detail. At first glance, you may think that there is nothing to discuss since open source are free and close source are paid. But in reality, you have to spend money in both cases.
While free to use, open source AI may require more technical expertise to customize and integrate into your existing systems. The costs associated with hiring or training developers to work with open source tools can be significant. Additionally, ongoing maintenance and updates depend on your team’s capability, which could lead to higher operational costs over time.
Closed source AI solutions usually come with dedicated support and are often easier to implement, reducing the need for deep technical expertise. However, any customizations may require additional payments or might not be available, potentially limiting flexibility and increasing dependency on the vendor.
In the long run, closed source AI typically includes ongoing updates, security patches, and technical support, which could reduce long-term operational costs. However, these savings are offset by recurring licensing fees, which can become substantial over time.
Another factor that impacts costs is data. Closed source models often come with access to proprietary datasets, particularly in specialized domains. The cost of these models includes access to these valuable datasets, which can be a significant advantage if your project requires such data. With open source models, in contrast, the costs of acquiring and integrating proprietary datasets or highly specialized data can be prohibitive.
When choosing between open source and closed source AI, consider the balance between initial cost savings and long-term financial commitments. Open source AI is ideal for organizations with limited budgets and technical expertise, offering lower initial costs but potentially higher customization and maintenance expenses. Closed source AI, while more expensive upfront, can provide valuable support, specialized data, and ease of scalability, making it a better option for enterprises with larger budgets and specific needs.
Security
As we’ve mentioned, closed source AI models are generally considered more secure. However, the overall answer to whether you should choose closed source or open source AI in terms of security is not that simple. It depends on the level of security your project requires. Even proprietary models can raise some security concerns. Let’s see why.
There are at least 10 common security risks you need to consider when building a project driven by LLMs, no matter whether proprietary or not. As closed source models are managed by a single organization, you have to trust in the vendor’s ability to handle security. If the provider doesn’t promptly address vulnerabilities or if their security practices aren’t up to par, it can pose significant risks. For instance, while it’s convenient that closed source models give you access to their extensive databases, these databases aren’t immune to a hack attack. Hackers could potentially “poison” the databases with malicious content, altering the data in a way that affects the AI’s output. Although the risk of this happening is generally lower with closed source AI compared to open source one, it’s still possible. This is why trusting your vendor’s security measures is crucial — if they slip up, your entire project is doomed to fail.
With open source models, on the other hand, a lot of people can review and catch security issues and suggest improvements. But you still need to be proactive about managing your own security. You’ll have to be on the lookout for fresh updates and possibly implement some custom security measures. While the open source community can be a great help, the responsibility for keeping the system safe largely falls on your shoulders.
This is where having a dedicated team of specialists becomes crucial. You’ll need experts who can actively manage the process, regularly update the model, and implement the necessary security protocols. Without a proper team, the risks can quickly outweigh the benefits of using an open source model.
Why settle for one when you can have both?
Both open source and closed source AI models have their strengths, and the right choice depends on your business's strategic goals, industry requirements, and operational needs. But honestly, you don’t have to pick just one — mixing both open and closed source AI can be a game-changer. Let's say you’re running an e-commerce web platform, for example. You can integrate PyTorch’s recommendation engine (which is open source) with IBM Watson Assistant (closed source) to improve customer experience. Suppose, a customer asks about recommendations for running shoes. IBM Watson Assistant handles the initial query and gathers information about the customer’s preferences. It then passes this information to the PyTorch recommendation engine, which analyzes the data and provides tailored suggestions. Watson Assistant presents these recommendations to the customer, creating a seamless and personalized shopping experience.
Sounds great, right? But putting together a system like that isn’t something you can just make on a whim. It’s crucial to get professional help. When it comes to AI, there’s rarely just one “right” solution, especially given the variety of models and tools out there. And it’s not just about using well-known AI models. Sometimes, you need to create custom machine learning algorithms (like we did for CV Compiler) or tweak pre-built AI tools.
With a solid track record of successful projects, we at Flyaps know how to navigate the complexities of AI. We can evaluate all possible solutions — some that might not even cross the minds of non-technical people — and guide you toward the best approach for your business. Whether it’s finding the right mix of open source and closed source models or developing something entirely custom, we’re here to make sure you get the results you’re aiming for.
Interested? Drop us a line to get started!
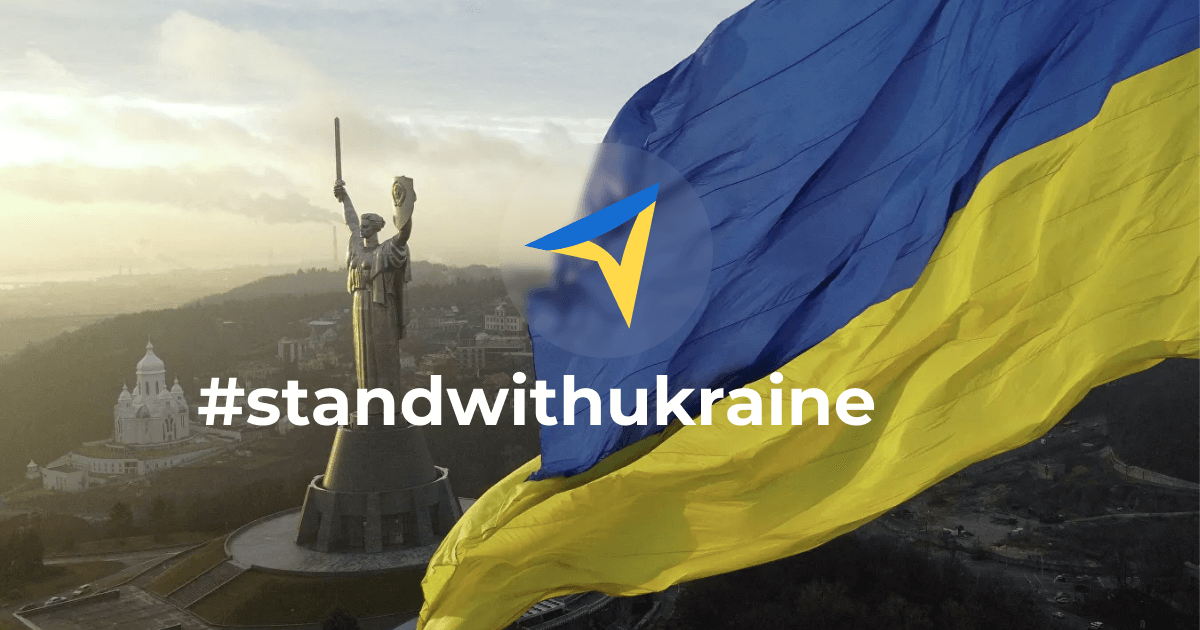