Telecom Data Analytics: 7 Real-Life Use Cases of Improving Operations with Big Data Analysis
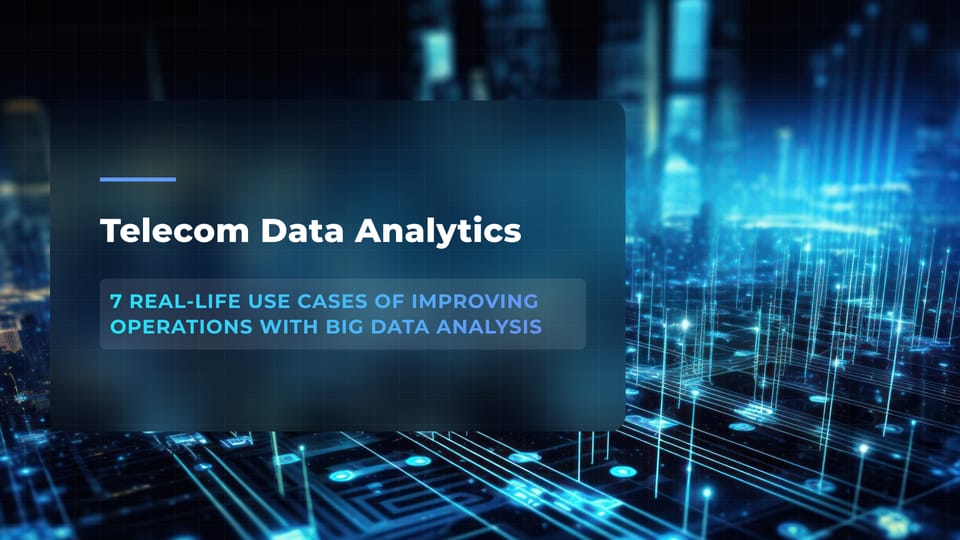
Telecom companies are in a tough spot right now. High customer churn, difficulty in identifying new revenue streams, and the constant need to optimize networks are just a few issues that block telecom growth and revenue.
But here’s the good news. All these challenges can be solved if you know how to use your data. Mobile devices, IoT, and network traffic have created millions of possibilities to use anonymized datasets. Telecom service providers can extract actionable insights about customer preferences, discover new revenue streams, and come up with better network usage from this vast information pool. Global telecom companies have already recognized the value of their data and as a result, the global big data analytics market is projected to skyrocket from $4.91 billion in 2024 to an astounding $155.33 billion by 2031.
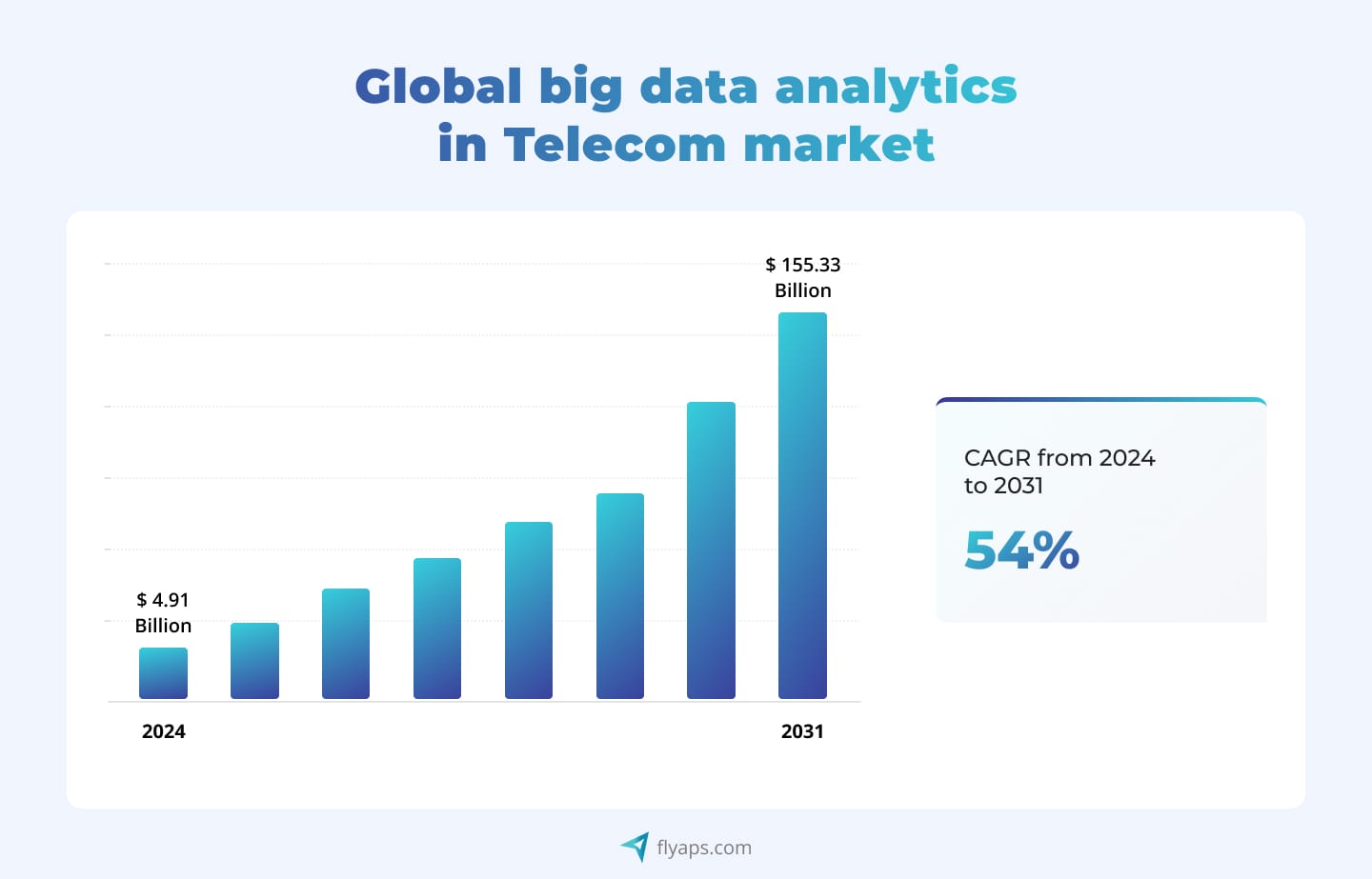
At Flyaps, we’ve been working in the telecom industry for more than 10 years now, creating custom solutions for global providers like Yaana and Netspark IP & Telecom and building our own AI/ML models for big data analysis. In this article, we’ll examine real-life telecom analytics use cases to see how telcos can use their data to get valuable insights, enhance operations, and improve revenue.
7 telecom analytics use cases you can learn from
Let’s take a look at seven examples of telecom companies that have already applied big data analytics and managed to enhance their business operations.
Use case #1: Enhancing customer experience and retention
Telecom is considered one of the industries with the highest customer churn. Users switch providers each month for mixed reasons, be it poor service, billing disputes, or just because they simply found a telecom provider offering a better deal. All of it makes customer retention quite challenging.
Data analytics help solve this problem by analyzing large amounts of anonymized data and extracting valuable insights from it. Analyzing data like customer behavior, call drop rates, and usage patterns helps telecom providers come up with personalized offers to address client complaints and improve the retention rate.
Global providers are already seeing the benefits of telecom analytics. Take Vodafone, for example. They teamed up with Google Cloud and Quantexa to develop a unified system that gives them a 360-degree view of their customers. Here’s how this system works.
Vodafone improves customer experience with Quantexa and Google Cloud.
Using Quantexa’s AI-based platform, Vodafone can standardize, enrich, and de-duplicate data, providing real-time insights into customer interactions and product usage. As a result, Vodafone can offer personalized deals for their clients, predict churn, and step in before a customer decides to leave. As a result, Vodafone benefits from happier customers and stronger, long-term loyalty.
Use case #2: Improving data monetization
Another problem many telecom companies face is identifying new revenue streams. Traditional sources like voice and messaging services no longer bring the expected revenue due to the rise of services like WhatsApp and Zoom, which have significantly reduced the demand for traditional communication services. Telecom companies must explore new business models to generate fresh income.
And here’s when big data for telecom analytics becomes a great advantage. Studying customer behavior, usage patterns, and emerging trends not only enhances telecom’s existing offerings but also opens up opportunities for monetization. After processing, this data holds a value that extends beyond the telecom industry itself. Telcos can offer valuable insights on existing trends to other sectors like healthcare, financial services, or advertising without compromising user privacy.
Deutsche Telekom's T Business initiative provides a great example of data monetization through the use of big data analytics. One of its core platforms, the Data Intelligence Hub (DIH), allows businesses to securely exchange, process, and analyze both internal and external data sources.
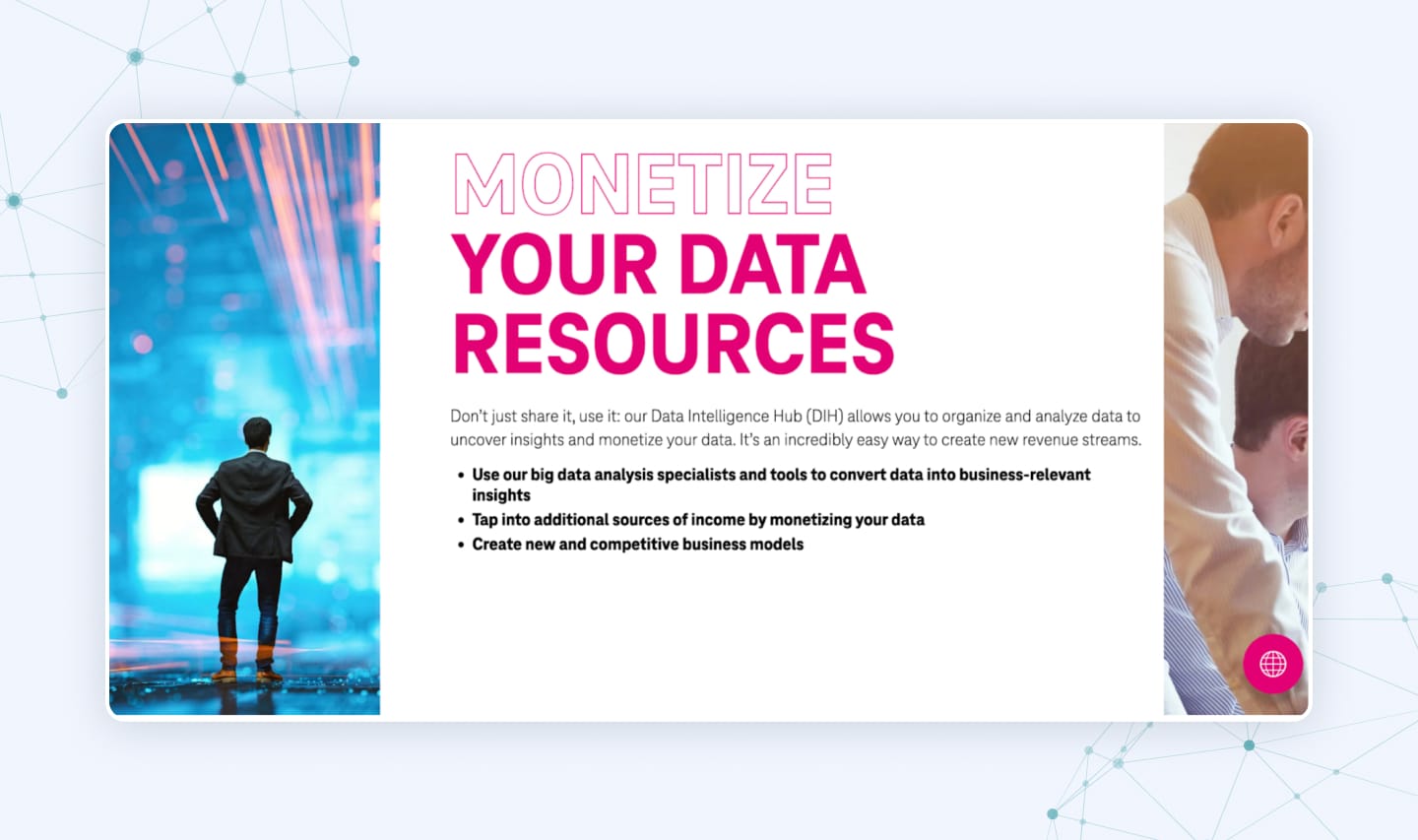
Businesses can use DIH to access over a million data sources, apply AI-driven analytics tools, and securely manage their data for maximum value. By integrating big data analytics, Deutsche Telekom gives companies a chance to use data as a strategic asset, driving revenue through insights, improving products, and opening up new income streams.
Use case #3: Fraud detection
According to the Communications Fraud Control Association (CFCA), telecommunications fraud increased by 12% in 2023, resulting in an estimated $38.95 billion in losses. The most frequent frauds include identity theft, subscription fraud, and unauthorized network access. Such attacks not only drain resources but also undermine customer trust and damage a company’s reputation.
Big data analytics can help solve this problem and improve the company’s resistance to fraudulent actions. Advanced analytics allow telcos to detect patterns and behaviors indicative of fraudulent activities by analyzing vast amounts of data in real time. For example, analytics systems can recognize phrases commonly used by cybercriminals, flag unusual activity patterns, and intercept fraudulent calls and messages before they reach customers. Machine learning models continuously improve by learning from new data, making them increasingly effective at identifying and preventing fraud.
China Mobile Communications Corp, one of the world’s largest mobile carriers, decided to act against telecom fraud by developing an advanced anti-fraud system called Tiandun (Sky Shield). Sky Shield is trained on a massive dataset of fraud cases provided by local police, enabling it to recognize suspicious communication behaviors that are common in fraud cases. The system can distinguish fraudulent calls and messages from normal ones, automatically intercepting spam and preventing it from reaching customers.
This targeted use of big data analytics and AI has allowed China Mobile to significantly reduce instances of fraud, protecting both their revenue and their customers’ trust.
Use case #4: Network optimization
Network optimization remains a critical challenge for telecom companies, especially as 5G networks expand and data consumption soars. With millions of connected devices and increasing bandwidth demands, telcos must ensure their networks can handle the load without compromising quality or speed.
Big data analytics help solve this challenge. Predictive analytics can forecast where and when future demand will spike, allowing operators to optimize their resources, reroute traffic, and prevent congestion before it even starts. These analytics tools process data from various sources, including network equipment, user devices, and environmental factors, to create a comprehensive picture of network usage.
AT&T is a compelling example of how big data analytics can enhance network performance and customer satisfaction. Over the past six years, the amount of data on AT&T’s network has skyrocketed by an astonishing 50,000%. In response to this explosive growth, AT&T has turned to big data analytics to make sense of this vast amount of data. AT&T Labs developed advanced analytics technologies, including the Tower Outage and Network Analyzer (TONA). This tool uses real-time and historical data to assess the ripple effects of network disruptions, guiding repair teams to prioritize cell towers based on their impact on customers.
Here’s a short description of how it works:
TONA has significantly improved AT&T's ability to identify customer impacts, achieving a 59% enhancement in decision-making for network issues.
Use case #5: Price optimization
Setting the right price for telecom services is a complex process, to say the least. On the one hand, telcos need to stay competitive in a crowded market where customers are always looking for the best deals. On the other hand, they have to cover their operational costs and ensure profitability. The problem is that customer preferences are anything but uniform. What works for one segment might not appeal to another. Additionally, there’s constant pressure from competitors who lower their prices.
Here’s how big data analytics can solve this problem. AI-powered systems can analyze customer behavior, usage patterns, and market trends, allowing telecom companies to gain insights into what different customer segments value most. This helps them create tailored pricing strategies that resonate with specific groups, offering just the right mix of services at a price point that feels right to the customer while still being profitable. Analytics also helps telcos keep an eye on the competition, enabling them to adjust prices dynamically based on market shifts, ensuring they stay competitive without sacrificing their bottom line.
Telcos can implement dynamic pricing strategies using software like PROS Pricing Solutions. PROS uses AI and machine learning to analyze customer data, predict how customers will respond to different pricing models, and automatically adjust prices to match demand.
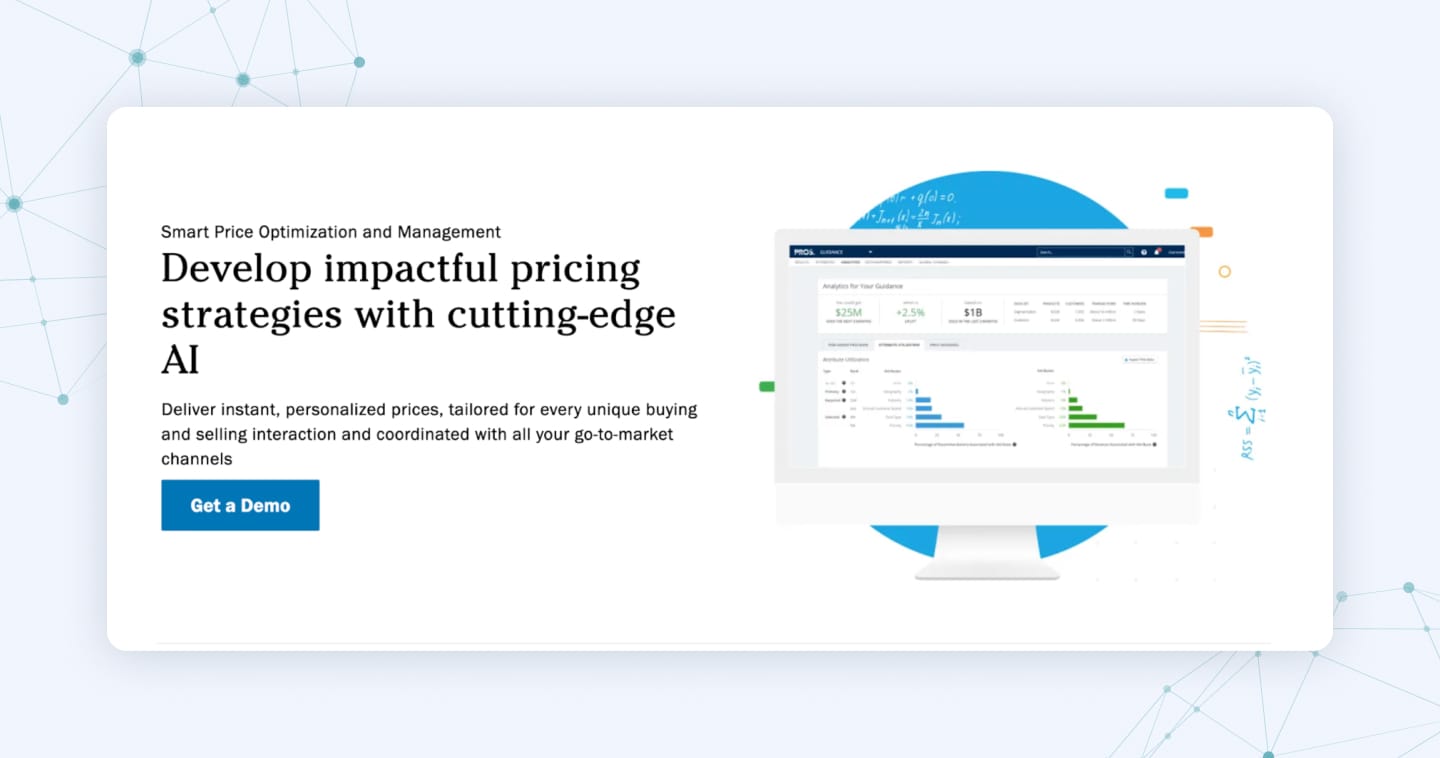
For instance, if the software detects that a competitor has lowered prices on a similar plan, it can automatically adjust prices or offer additional value, like bundling services, to retain customers. Additionally, PROS can identify opportunities to upsell or cross-sell services to high-value customers, increasing average revenue per user (ARPU).
Use case #6: Operations analysis
Big data analytics can improve operations analysis by aggregating data from various sources, such as network performance, customer interactions, and operational workflows. With these insights, telecoms can identify inefficiencies, forecast demand, and streamline processes.
A great example of using data analytics in the telecom industry for operations analysis is Vodafone. Datatonic built for Vodafone an ML-powered system that forecasts internet traffic on a mobile network, using anonymised open-source data.
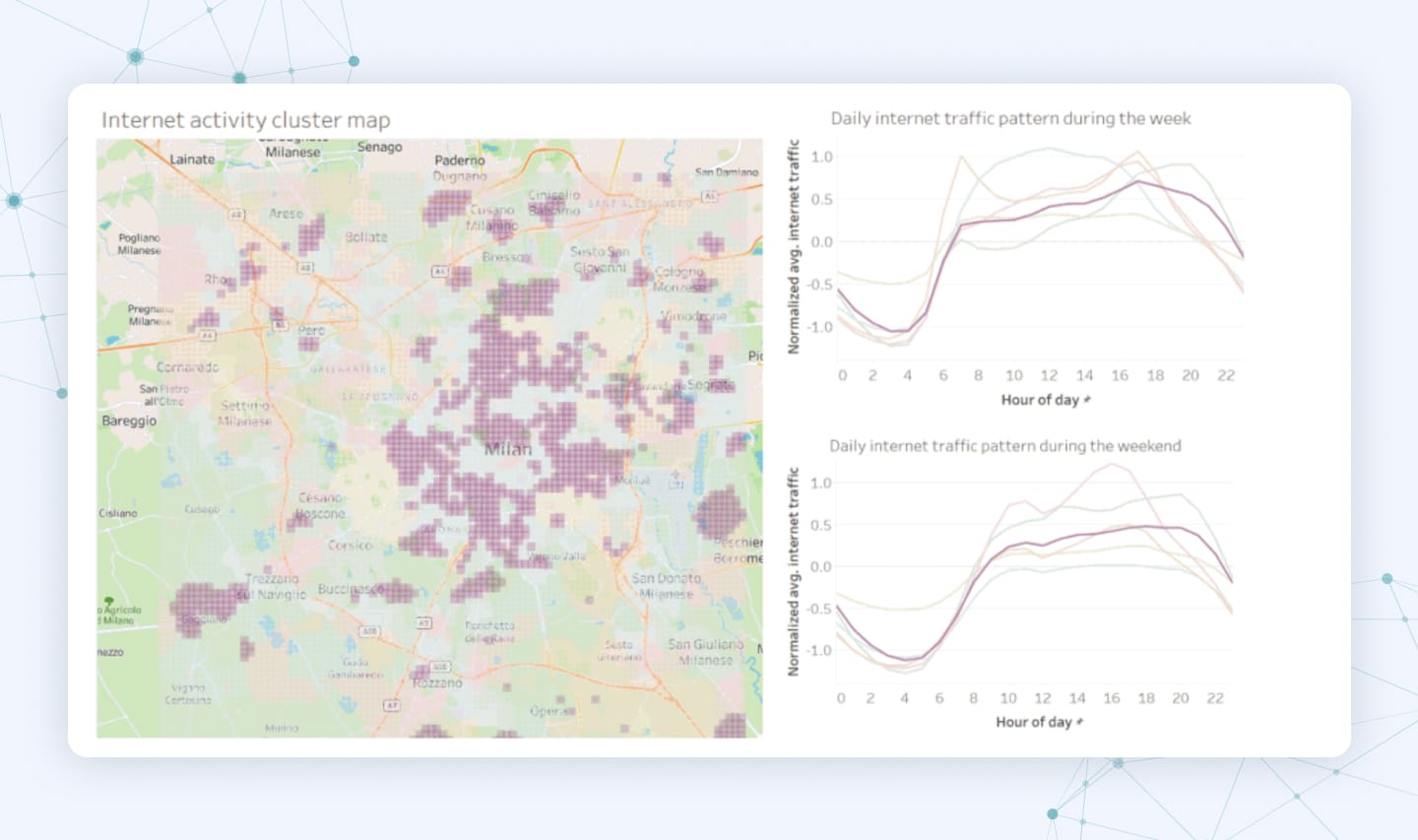
As for the results, Vodafone managed to significantly improve its energy efficiency by analyzing network usage patterns. The data showed when certain network components were underused, enabling Vodafone to power down parts of the network during off-peak hours. Vodafone also uses real-time analytics to monitor energy usage and deploy energy-saving software across its mobile base stations, cutting costs and contributing to sustainability.
Vodafone example showcases how telecom data analytics can have a direct impact on both cost efficiency and customer satisfaction.
Use case #7: Optimizing roaming revenue
MNOs often struggle to effectively manage their wholesale and retail roaming businesses. They face difficulties in optimizing revenue, negotiating favorable discount agreements with partners, and predicting usage patterns, all of which can impact their profitability.
A customized analytics platform can empower MNOs to analyze, simulate, and predict future revenue and margins associated with roaming services. By leveraging big data analytics, telecom companies gain insights into customer roaming behaviors, assess partnership performance, and fine-tune pricing strategies.
Based on our experience, we worked with one of the largest clearing companies to transform their OSS/BSS system and upgrade their analytics software for optimizing roaming revenue. The system now delivers dynamic dashboards that organize insights by country, service type, and other key metrics.
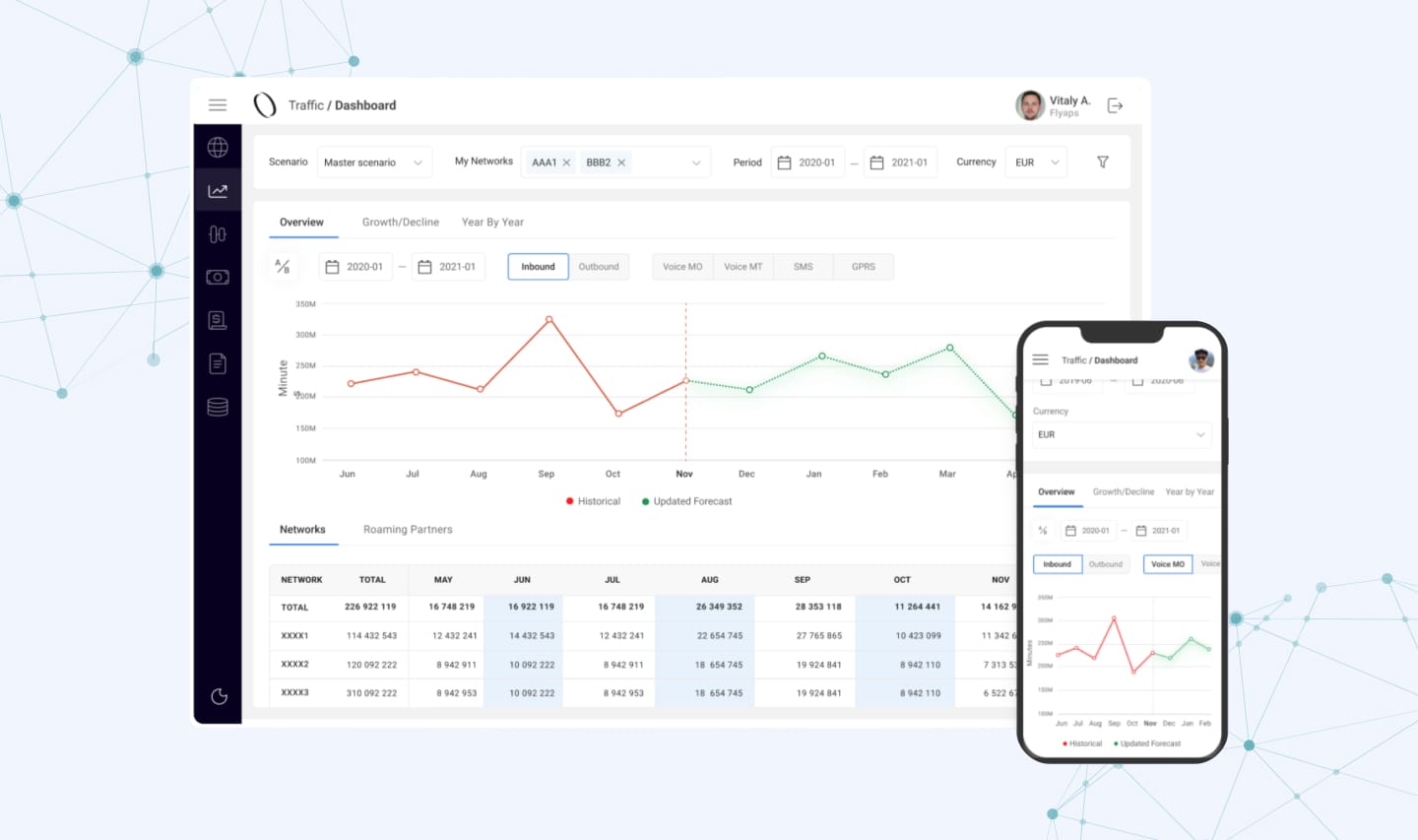
The platform we delivered lets MNOs analyze traffic volume, inter-operator tariff rates, and discount agreements. It features a budget module that enables users to create and compare multiple budgets, identifying the most profitable options. The solution is now used by major companies like Orange Group and Hutchison 3G UK Ltd., helping them forecast, budget, and analyze roaming data effectively.
We analyzed the main use cases for telecom data analytics but how to successfully adopt them and achieve the same results? First, telecom companies need to gather data and get insights that would allow them to make informed decisions. This process involves several frequent challenges that telcos need to consider before implementing a data analytics system.
Key challenges companies face in implementing telecom data analytics
Here are the main challenges companies face when adopting big data analytics in telecom industry:
Data quality and preparation Diverse and siloed data sources Data privacy and security Skill and talent gap Inaccurate or inconsistent data can slow down the analysis process. Plus, traditional data systems lack flexibility, making it difficult to keep up with changing needs. Data from different channels, such as customer interactions and network metrics, often exists in varying formats and locations, creating silos that hinder analysis. Sensitive data makes telcos targets for cyberattacks. They need to protect privacy with security measures like data encryption, access controls, and regular security audits. As the demand for advanced data insights increases, there’s a shortage of professionals with strong skills in data science, machine learning, and analytics.
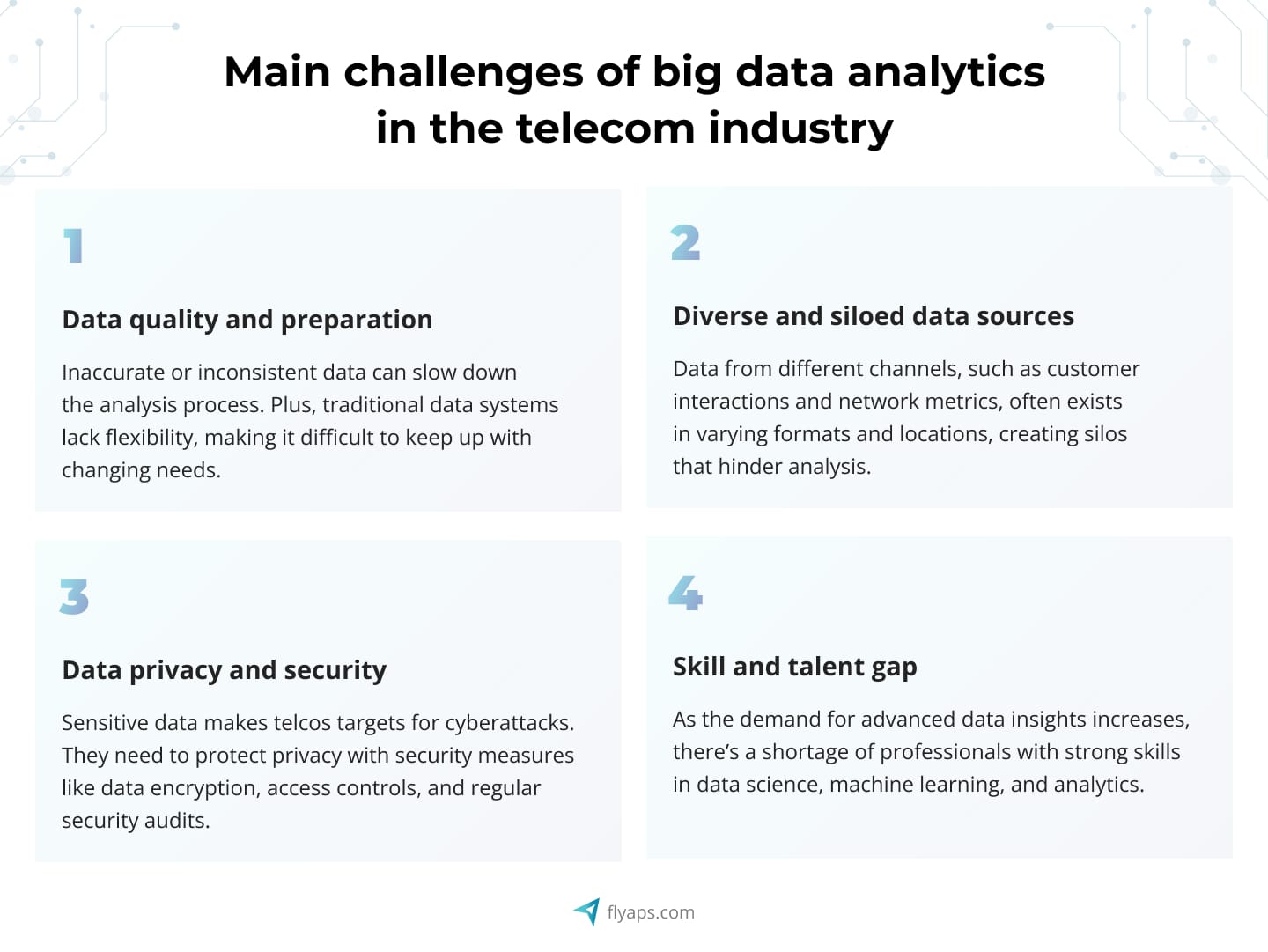
Now, how can big data analytics for telecom be applied to help companies solve these challenges and gain insights? One of the smartest ways to facilitate data analysis is to use generative AI. Let’s see how AI facilitates analytics in the telecom sector.
Generative AI in telecom data analytics
Generative AI can multiply the benefits of data analytics in the telecom industry by simplifying complex data analysis and making large datasets more accessible. Let’s take ChatGPT as an example. This Large Language Model (LLM)-powered assistant analyzes vast public datasets to quickly respond to the users’ prompts.
Similarly, telcos can build and train their own LLMs on a chosen dataset to enable it to generate clear, actionable insights from vast amounts of data. Pre-trained AI models can process queries swiftly, offering precise answers that enhance network operations, improve decision-making, and broaden data access across teams.
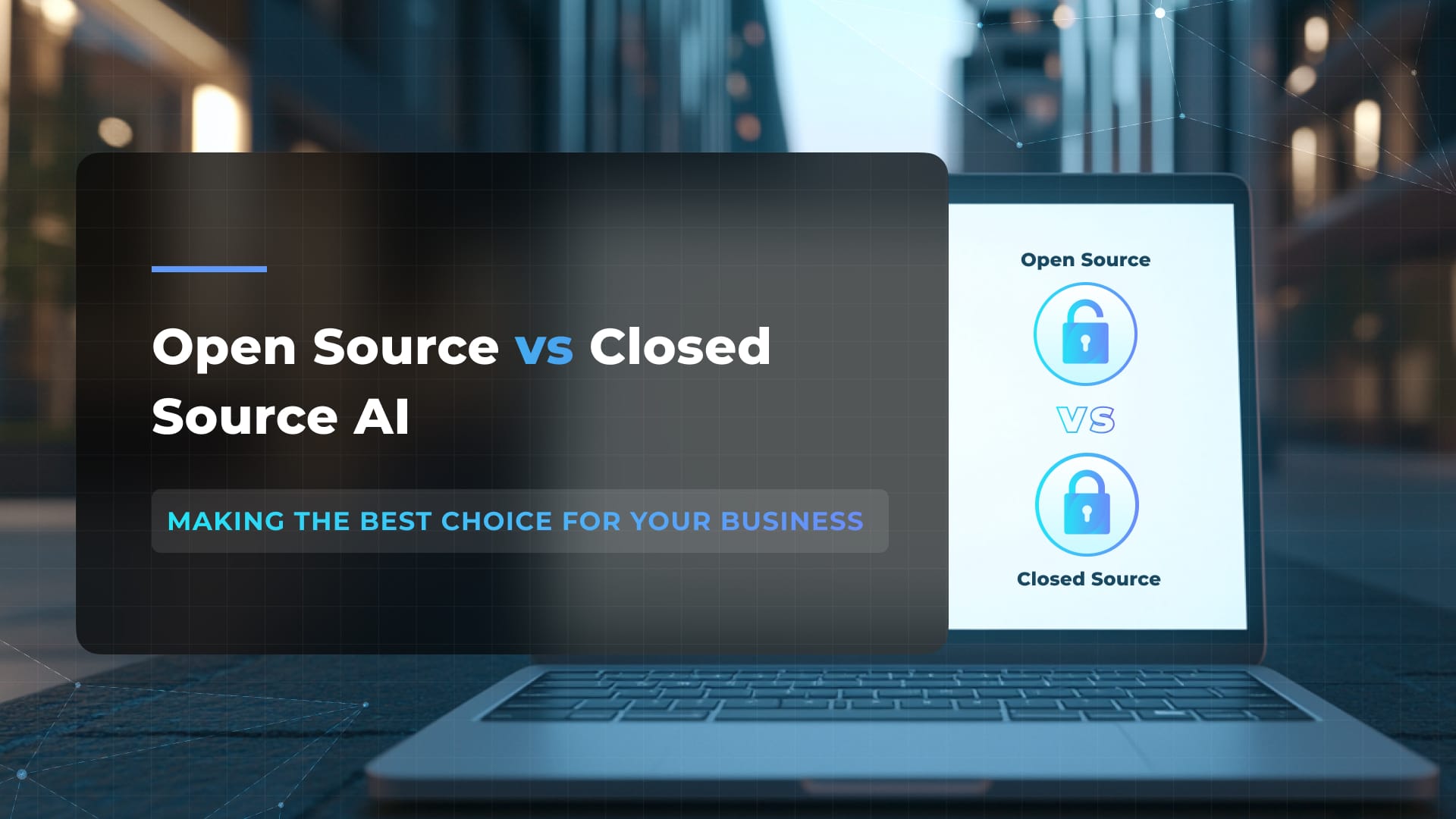
Check out our article about Generative AI in telecom for more details
For example, Vodafone's AI-driven virtual assistant, TOBi, handles a variety of customer service tasks across multiple countries. It operates in 15 different languages, helping customers with queries related to billing, technical support, and account management. TOBi can answer questions, provide service recommendations, and assist with solving common issues. By automating these routine tasks, TOBi significantly reduces wait times and improves the overall customer experience.
As Scott Petty, Chief Technology Officer of Vodafone, explains:
“We see AI primarily as a ‘virtual assistant’ for humans. Already AI is freeing-up Vodafone employee time from tedious, repetitive manual work, enabling them to focus on more creative activities that benefit our customers and the environment.”
In this video, Scott Petty shortly describes how Vodafone uses AI in 3 different ways to better analyze their data and improve the user experience while decreasing staff workload:
Overall, Generative AI offers significant opportunities for telecom big data analytics. Global companies like Vodafone are already actively using it for different goals, from customer support to operations automation.
Bottom line
As we've seen through the various telecom analytics use cases, from improving data monetization to optimizing roaming revenue, big data analytics are changing the telecom industry. But to fully benefit from these new opportunities, providers must invest in a comprehensive telecom data analytics solution that integrates data from diverse sources.
At Flyaps, we have practical experience of building custom telecom solutions from scratch. Beyond that, we offer tailored solutions built on AI/ML models specifically for telecom companies that want to improve their operations with big data analytics fast and cost-efficiently.
If you want to benefit from AI 3X faster with pre-built solutions or need a custom telecom product, contact us, and we’ll be back to you soon to discuss how we can help you meet your needs and goals.
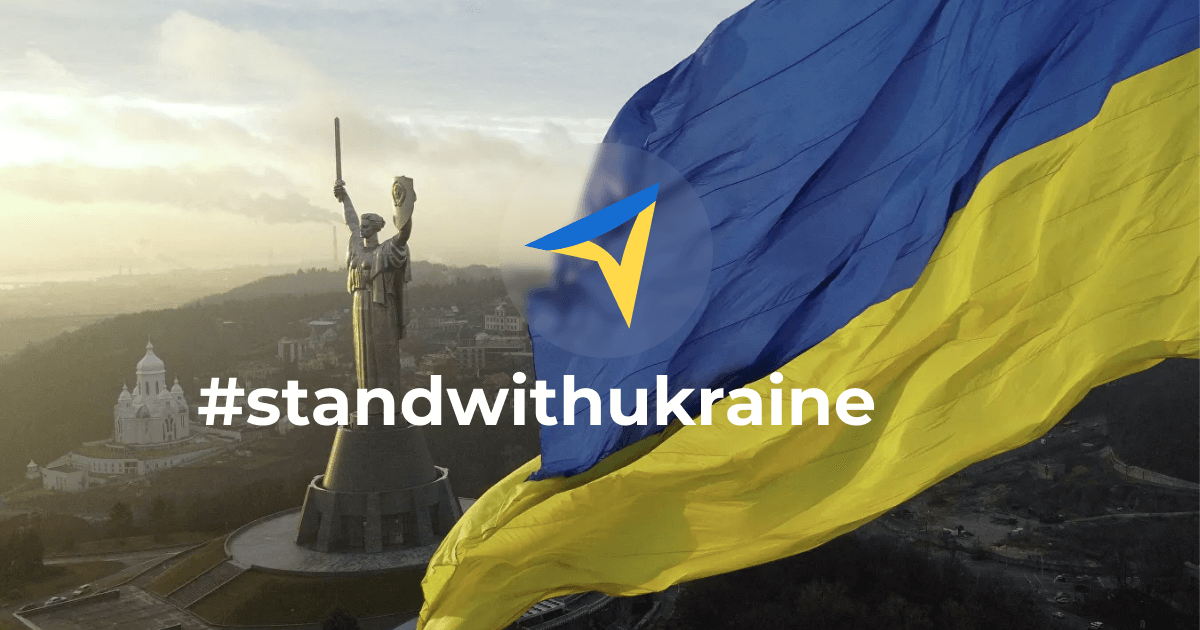
FAQ
What are telecom analytics use cases?
Telecom analytics use cases include improving customer retention, detecting fraud, and optimizing network performance for better resource allocation.
What challenges do telecom companies face in implementing data analytics?
Common challenges include ensuring data quality, managing diverse and siloed data sources, addressing data privacy and security concerns, and overcoming skill gaps in data science and analytics.
How is generative AI used in telecom data analytics?
Generative AI simplifies complex data analysis by enabling telcos to generate actionable insights from large datasets. For instance, AI-driven virtual assistants can automate customer support, decreasing employee workload and helping with common issues.
What is the future of big data analytics in the telecom sector?
The future of big data analytics in telecommunication looks promising, with the global market projected to grow significantly. As telecom companies increasingly recognize the value of their data, they will continue to use analytics for operational improvements.