Understanding Data Mining in Telecom: What Knowledge Is Buried Within Data Sets?
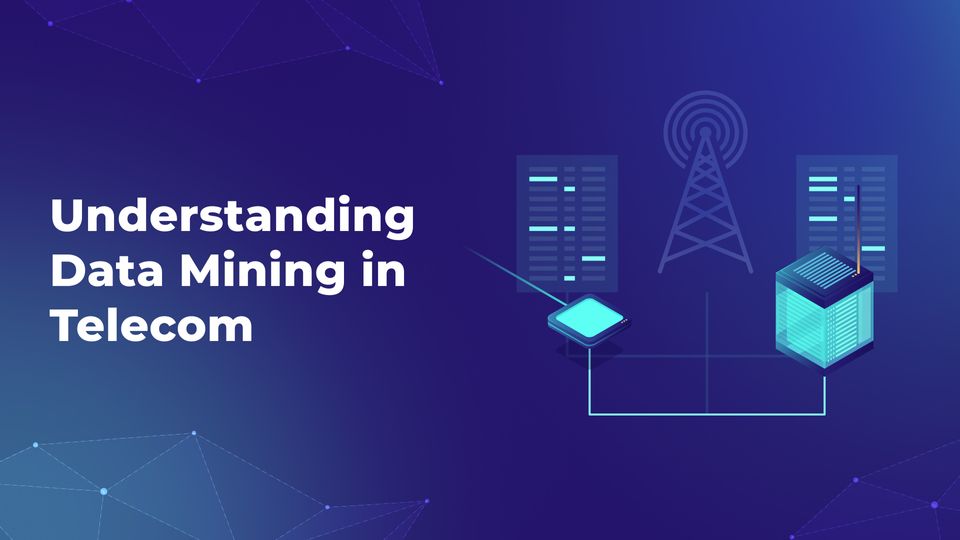
The world of telecommunications has a lot of data to work with. Service usage, network performance, customer data – we’re talking about terabytes if not petabytes of data generated every single day. So eventually, every telco faces a challenge: how to make sense of all this data? Thankfully, there’s an answer and that is data mining.
In fact, data mining isn't just for telecom. It has become a popular practice across industries, delivering impressive results. A staggering 90% of companies engaged in mining big data are reaping significant rewards. Experts believe that data collection, analysis, and interpretation have the potential to revolutionize all kinds of businesses.
At Flyaps, we've been partnering with telcos around the globe for ten years. During this time, we've noticed a rising trend in data analytics solutions. We've even developed numerous platforms ourselves to support data analytics for our clients. These solutions helped them transform their businesses and gain valuable insights into customer behavior, enhance network performance, and make intelligent decisions using data.
In this article, we want to share our piece of knowledge about data mining in the telecom industry. We'll also explore how adopting data mining can benefit your business and empower you with valuable information, so keep reading to learn more.
Three main types of data in telecom and why mining them
Let’s start with data types. In the field of telecommunications, there are three main types of B2B and B2C data that are considered the most crucial ones. They are call-detail data, network data, and customer data. Let's explore each of them and understand why mining these types of data is essential.
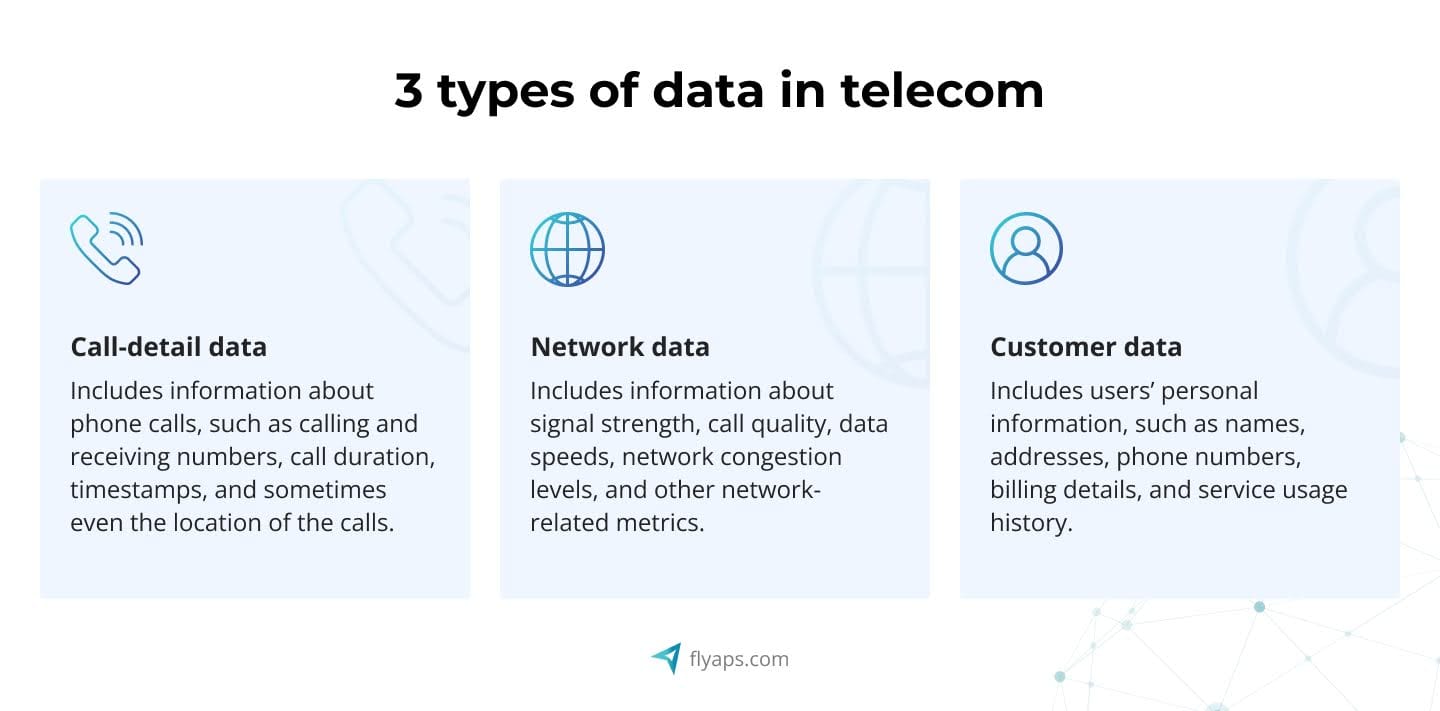
Call-detail data
Telcos have a lot of information about the phone calls their customers make. This data includes things like the numbers people call and receive calls from, how long the calls lasted, when the calls were made, and sometimes even where the calls took place.
By looking closely at all this call information, a telco can learn some interesting things. For example, they can find out which places their customers call the most. Maybe some clients have customers or partners in specific cities or countries. This information in the data mining process helps to understand the calling patterns and preferences of users of telecom services.
Telcos can also figure out the busiest times for calls. For instance, using data mining businesses can learn that most of their customers make a lot of calls in the morning when they're scheduling meetings or discussing important matters. By knowing the busiest times, telcos can ensure that their phone lines are well-equipped to handle the increased call volume, preventing any delays or call quality issues.
And here's the most exciting part: telcos can use all this information in the data mining process to improve customers loyalty. For example, with data mining, telcos can learn that many of their clients call a specific country a lot, and offer calling plans that fit their customers' preferences and let them save costs. Personalized offers make users understand that the telco they chose does care about them, so they want to stick around and enjoy the benefits.
From cloud migration to telecom-specific AI solutions, we have delivered over 20 projects that are used by hundreds of MNOs and telecom companies worldwide. Check our capabilities and let’s discuss your next solution.
See our servicesNetwork data
Network data tells you about the signal strength, call quality, data speeds, network congestion levels, and other important metrics related to the network.
By analyzing network data, telcos can identify any bottlenecks or issues in their network. For example, a telecom provider might discover that certain areas have low signal strength or experience frequent congestion during specific times of the day. This knowledge allows them to troubleshoot and take necessary steps to improve network performance, ensuring a smoother and faster internet experience for their business customers.
Furthermore, by mining network data, the company can predict network usage patterns. They might observe that during business hours, as an example, there is a high demand for bandwidth due to increased online activities. Armed with this information, they can optimize their network infrastructure by allocating resources where they are needed the most, ensuring that businesses have sufficient bandwidth to operate efficiently.
Customer data
Customer data encompasses personal information, such as names, addresses, phone numbers, billing details, and service usage history.
By using customer data in the data mining process, telcos can gain insights into their customers' preferences, behavior, and demographics. For instance, they might find that certain industries require higher bandwidth or have specific communication needs. With this knowledge, they can offer personalized services that cater to the unique requirements of each business customer.
Additionally, customer data helps telcos identify potential churn risks. They can analyze usage patterns, billing details and customer feedback to identify businesses that may be at risk of going to the competitor. By proactively addressing users’ concerns or offering special incentives, telcos can improve customer retention and reduce churn rates.
How data mining in the telecom industry works
In the telecom industry, the data mining process is typically carried out through the structured life cycle consisting of six stages. These stages are often interdependent and involve moving back and forth depending on the results achieved. So, let's have a closer look at these stages:
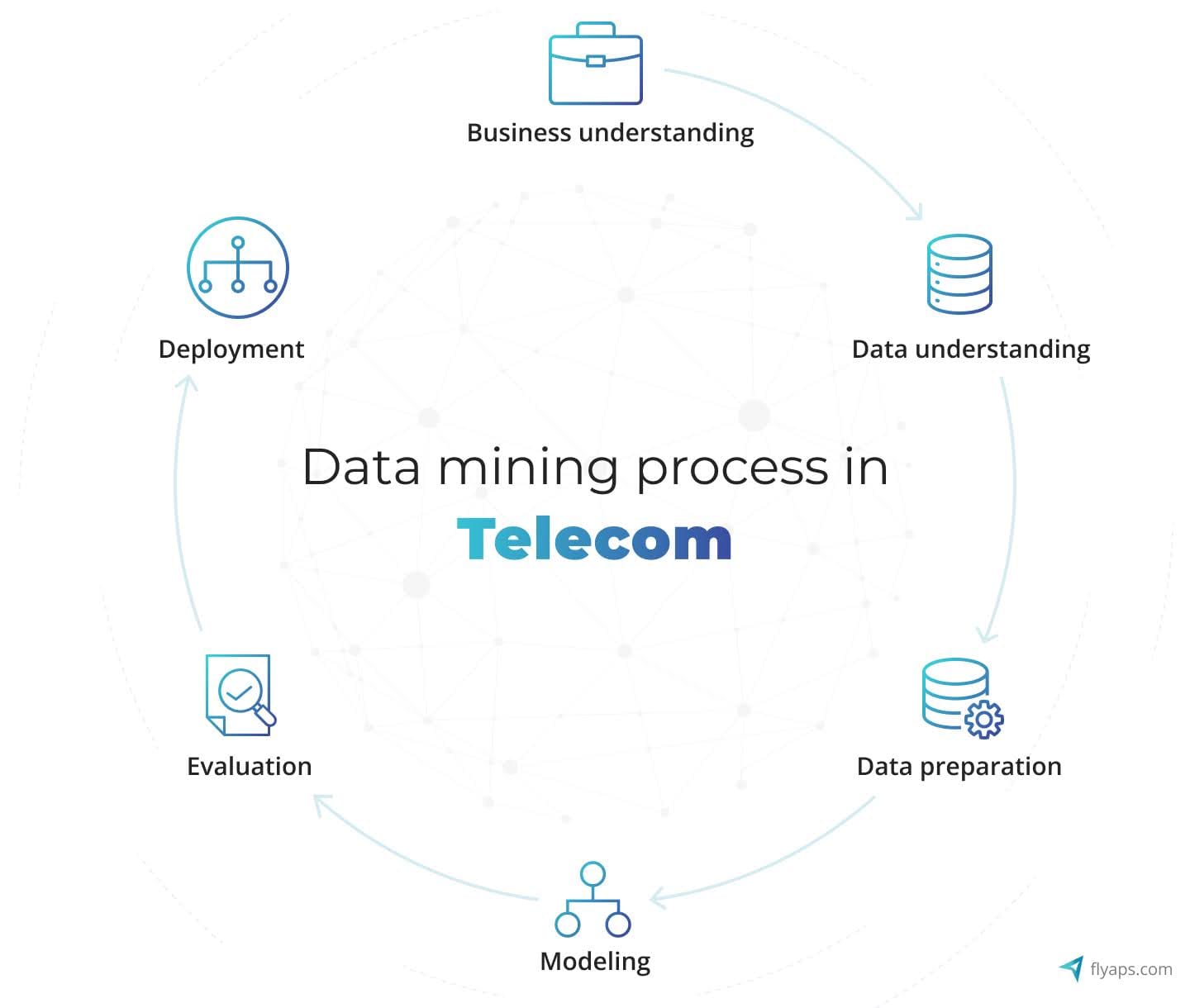
Business understanding
In this stage of the data mining process, you focus on defining the business requirements and project objectives. You gather information to define the data mining problem and create a preliminary plan to achieve your goals. In other words, you turn your business needs into specific data mining tasks.
Data understanding
Here, you start collecting and describing the initial dataset. You become familiar with the data, identify any issues related to data quality, and discover initial insights or interesting subsets that can help form hypotheses. This stage helps to gain a deeper understanding of the data you will be working with.
Data preparation
Data preparation in the data mining process involves activities like cleaning, transforming, and aggregating data to construct the final dataset. Here, you have to make sure that the data is in the right format, remove any inconsistencies or errors, and combine relevant data from various sources. You also prepare your data for the modeling stage.
Modeling
In this stage of the data mining process, you should apply various modeling techniques to the prepared data. These techniques can include clustering, classification, association rule mining, or predictive modeling. You adjust the parameters of the models to find the best values that yield accurate results. This step helps with uncovering patterns, relationships, or predictions within the gathered data.
Evaluation
Once the model is created, it undergoes a thorough review and evaluation. You execute the necessary steps to construct the model and ensure that it aligns with the business objectives. You assess its performance, accuracy, and reliability. Based on the evaluation results, you make decisions on whether the data mining results meet the desired outcomes.
Deployment
Data mining is required to gain knowledge from the data, and in this stage of the data mining process, you organize and present that knowledge in a usable way for customers. Deployment can be as simple as generating a report summarizing the findings, or as complex as implementing a repeatable data mining process across the entire organization. The goal is to make the gained insights accessible and actionable to improve decision-making and business operations.
To learn more about effective AI implementation in telecom and other industries, check out our article on AI Implementation strategies.
Data mining use cases for telecom
For better understanding of what results telcos can achieve by effectively collecting and using their big data, here are some case studies of data mining in telecommunications.
Cutting a customer churn rate by 50% with data mining
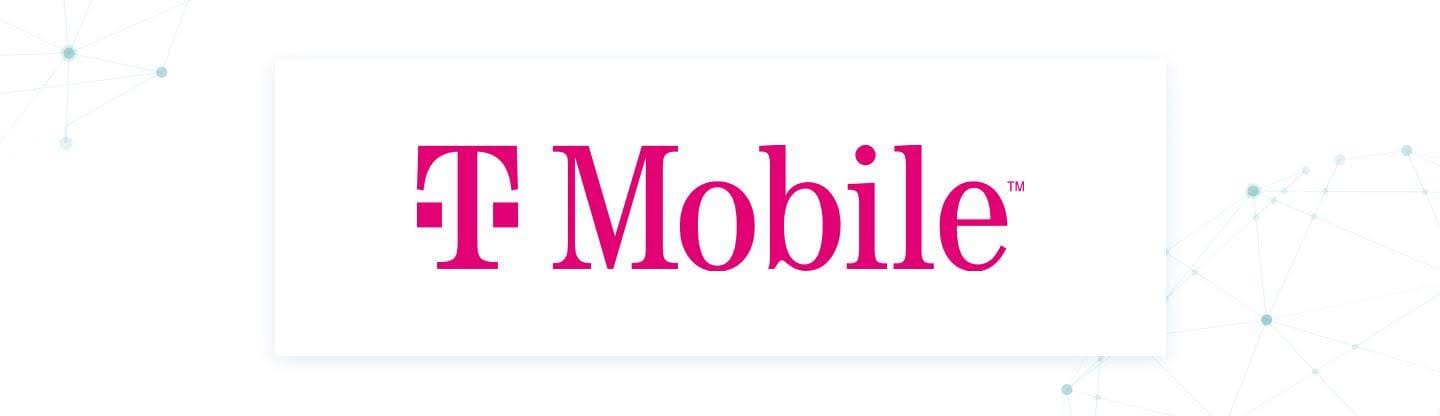
T-Mobile did something amazing with their big data strategy – they managed to reduce the number of customers leaving (churn rate) by a whopping 50%. Here’s how.
The company started by gathering all sorts of data about their customers and network. T-Mobile organized this data into six different zones, focusing on things like customer info, their experience with the company, and which products they used. Sounds complex, right? But it did help T-Mobile stay ahead of the game. By structuring data that way, T-Mobile was able to uncover trends in how people use their services, where they're calling from, and even how valuable each customer is.
Thanks to the data mining process they initiated, T-Mobile managed to see which customers have a strong social network and big influence on others. They could spot those people and give them special perks, like plan discounts. And when customers aren't happy, T-Mobile would take action. For example, if someone is using a lot of data on their tablet and complains it’s still not enough for them, they might get some extra data for free. And if someone's in the area with weak coverage, T-Mobile can quickly offer them a free gadget or even a new phone.
This personalized approach to customer care is what sets T-Mobile apart. Loyal customers get treated extra well without even asking. And if someone seems unhappy, T-Mobile fixes things before the problem even pops up.
As a result, T-Mobile added a staggering 2.1 million customers in just three months, and they've been growing steadily for a while now.
AI + big data usage by the UK telecom giant
Example of British Telecom (BT), the big telecommunications company in the UK, is one of the most famous data mining use cases for telecom. Let’s explore how they are using big data and artificial intelligence (AI) to improve their operations.
BT has quite a lot of client-related data to work with, and their goal is to give their customers a great experience and outshine their competitors. One way they do this is by using AI development platforms to build and deploy models that predict when there might be problems with their services. By analyzing all the data they have, they can figure out what might cause a service to stop working and then prioritize fixing it. This helps them plan resource allocation better and save a lot of money.
But BT doesn't stop there. They also work with other companies and use technology like data science and machine learning to predict how many calls their call centers will get. This allows BT to use their resources more efficiently and allocate their budget accordingly. As a result, the company is able to provide better customer service, while spending less money.
Another important thing BT does with AI is that they use it to protect themselves from cyberattacks. They built the Research Lab and Control Centre, where experts use AI for data analysis and finding any suspicious activities to fight against cyber attacks. This way, BT can stop the attacks before they cause any harm and keep their network safe and secure.
Top data mining techniques to use
Let’s look at how different data mining techniques in the telecommunication industry can be applied to gain valuable insights, enhance customer experience, and optimize business operations.
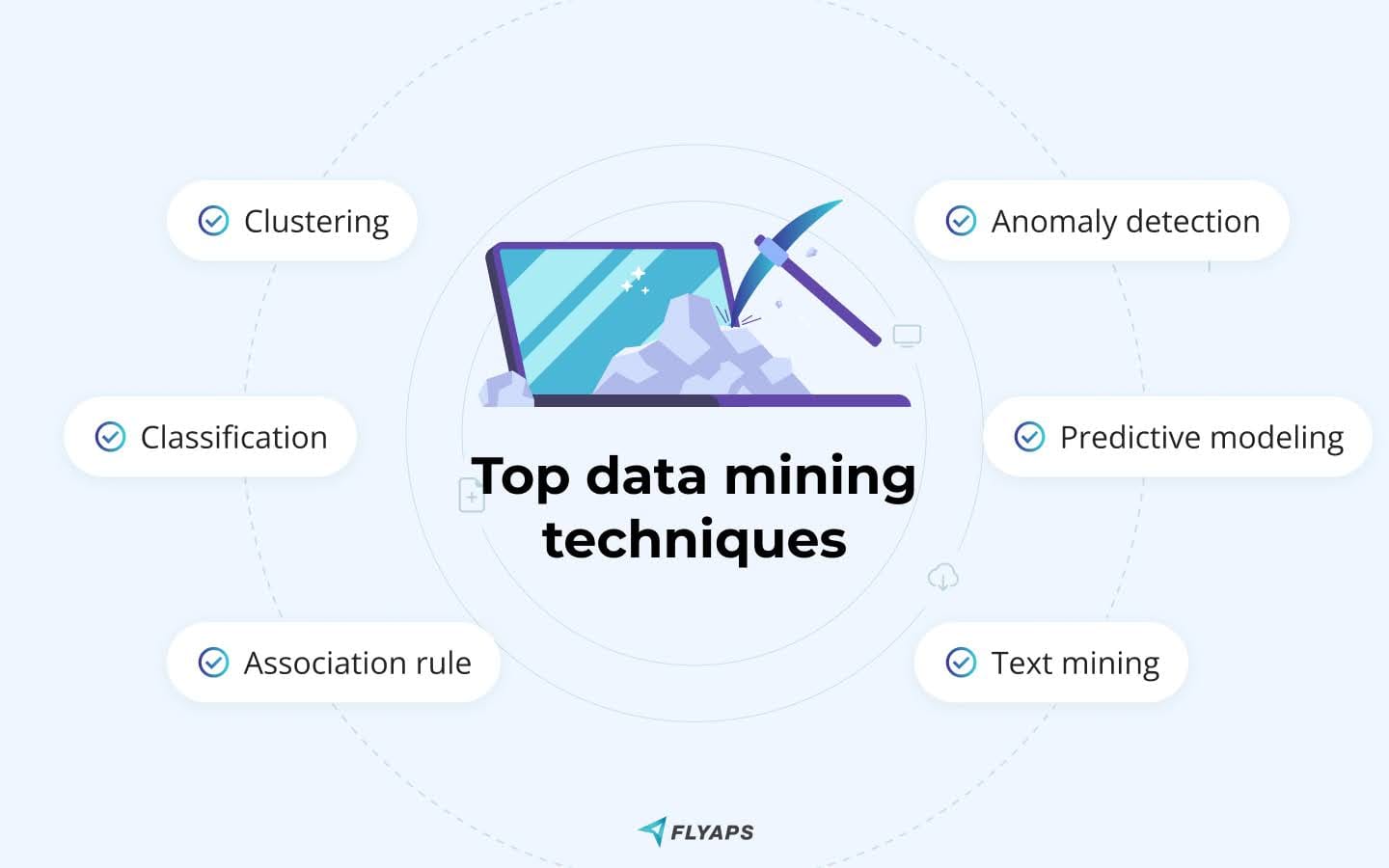
Clustering
Clustering can be applied to customer segmentation. Let's say a telecom provider wants to identify groups of customers with similar behaviors. By analyzing data such as call duration, data usage, and service subscriptions, the custom-built clustering algorithm can help identify different customer segments, for instance, "Frequent Internet Users" and "Frequent Callers." By splitting the target audience into segments, the company can create targeted marketing campaigns and personalized offerings for each group.
Classification
A telecom company can employ classification techniques for customer churn prediction. Using historical data, the classification algorithm learns patterns associated with customers who have previously switched to a competitor. Based on attributes like call duration, billing complaints, and service usage, the algorithm can categorize current customers into "Likely to Churn" and "Unlikely to Churn" groups.
Association rule
Telecom providers use association rule mining to identify cross-selling opportunities. Suppose the analysis of customer data reveals that customers who subscribe to a home internet service are more likely to also subscribe to a landline phone service. By leveraging this insight, the telecom company can create bundled packages combining both services and promote them to customers who have only subscribed to one of them. This strategy increases the chances of customers purchasing the additional service.
Anomaly detection
Anomaly detection techniques can be used for network security. Imagine a large telecom network where each user's communication patterns are tracked. An anomaly detection algorithm identifies a user who suddenly starts generating an unusually high amount of network traffic, which is far beyond their normal usage pattern. This discovery raises a security alert, as it may indicate a potential network intrusion or a distributed denial of service (DDoS) attack. The telecom company can then take immediate action to investigate and mitigate the issue.
Predictive modeling
Predictive modeling is employed by a telecom company to forecast network traffic. By analyzing historical data, such as peak usage times, seasonal variations, and new service launches, the predictive model can estimate future network traffic patterns. This information helps the telecom provider allocate resources effectively, plan network upgrades, and ensure optimal performance during high-demand periods.
Text mining
Telecom companies utilize text mining to extract insights from customer feedback. Imagine a telecom provider that receives a large volume of customer feedback through various channels, including social media, emails, and call center transcripts. By applying text mining techniques, the company can analyze the sentiments expressed by customers, identify common issues or complaints, and gain valuable insights to improve customer service.
Why choose Flyaps for your big data analytics
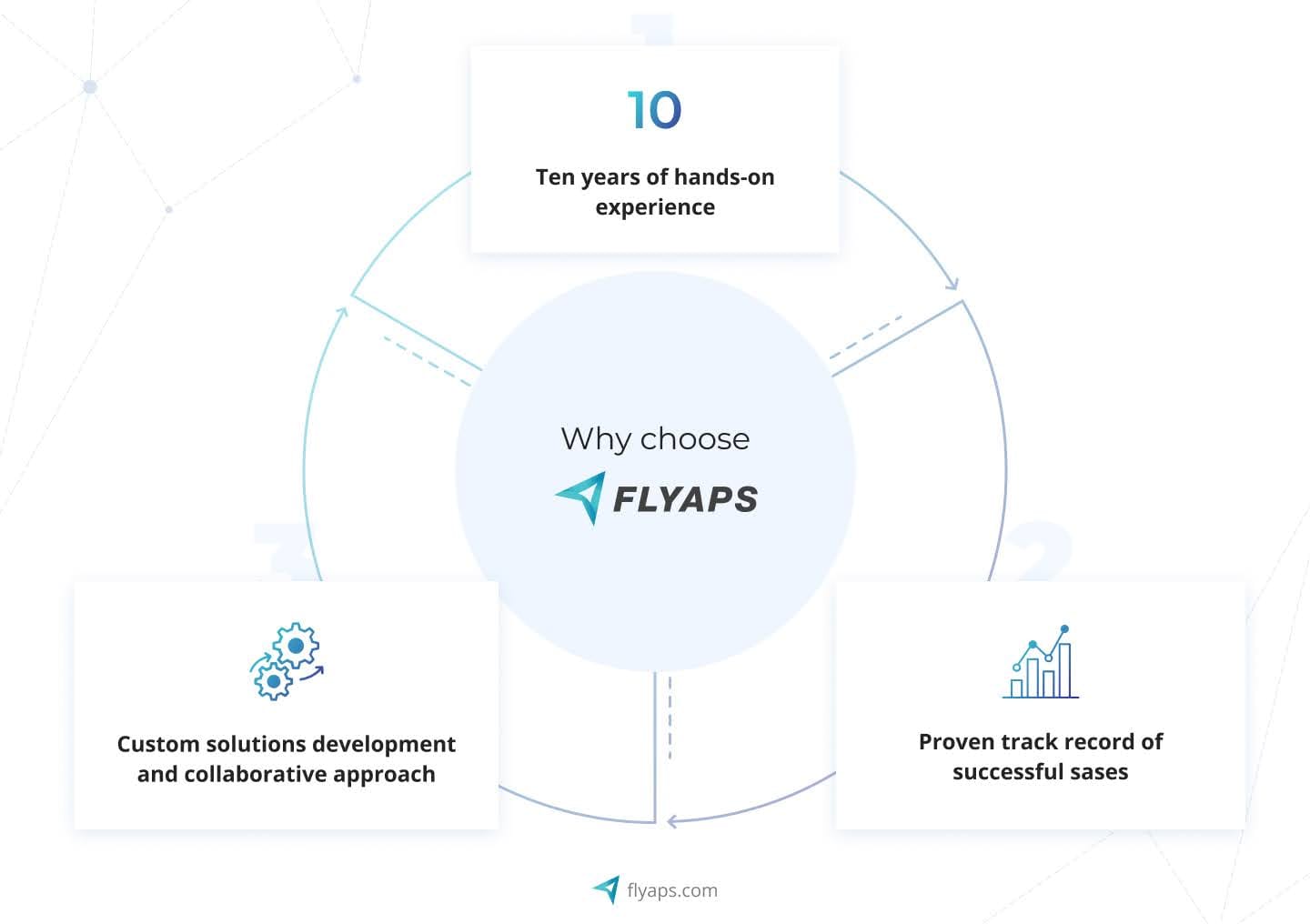
Ten years of hands-on experience: At Flyaps, we bring a decade of experience working closely with enterprises from the telecom industry. Our extensive knowledge and understanding of the unique challenges and intricacies in this sector make us a perfect fit for data mining projects.
Proven track record of successful projects: Our portfolio includes numerous successful data mining projects in the telecom industry. We have helped telecom companies unlock valuable insights, optimize network performance, streamline operations, and improve customer experiences through data-driven decision-making.
Custom solutions development and collaborative approach: Flyaps offers end-to-end data mining solutions tailored to our client's unique business needs. From data collection and cleansing to advanced analytics and predictive modeling, we provide a comprehensive suite of tools and methodologies. We prioritize a collaborative approach that puts you at the center of our work. We take the time to truly understand your unique requirements and involve you at every stage of the project, ensuring a tailored solution that meets your specific needs.Unlock the potential of data mining for your telco. Drop us a line today!